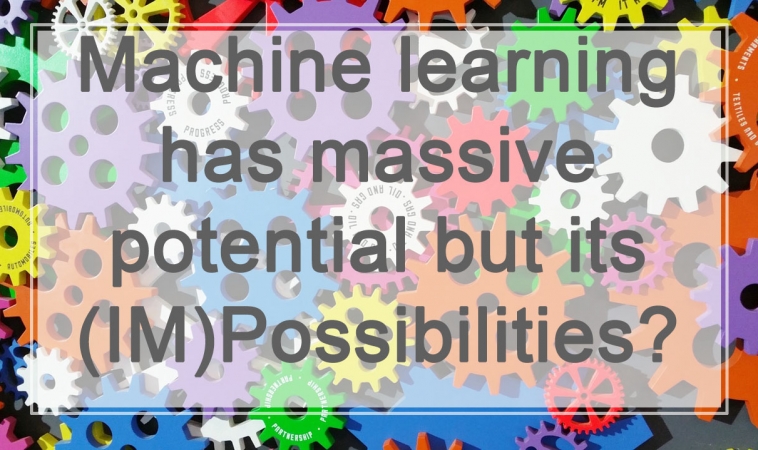
Contents
Machine learning has massive potential but its (IM)Possibilities?
Technology brings about a number of welcome advancements in human innovation. One of these such innovations is that of machine learning. Previously thought inconceivable, the ability of machines to gather, assimilate, and ‘learn’ has now become a possibility. This ability of machines to gather information has a lot of possibilities for humanity in different aspects, like finance, health and many more. However, with every prospect humanity brings comes a number of challenges. There are a lot of possibilities that come with the development of machine learning, but it is necessary to understand its shortcomings. Shining a light on these shortcomings or impossibilities is not to poke holes at this technology, but more to better understand the concept so we can further advance. In this articles, we would be taking a look at some of the impossibilities of machine learning in a modern world.
What are the impossibilities of machine learning?
– Assimilation but not activity
While machine learning has incredible abilities in terms of assimilation, one shortcoming it has and may continue to have for the foreseeable future (except if we begin to make unpredictable strides in artificial intelligence) is that computers do not have the ability to act upon this information without the human interference. This challenge transcends fields of just machine learning, but also affects artificial intelligence. Health companies for instance may be able to come up with the technology that is able to monitor and gather data on an individual’s lifestyle, health choices and other important aspects. However, these machines cannot come to make actions independently. This information has to still be acted upon by people. Assimilation is the bane of machine learning, but perhaps that may be all it is cut out to be. A welcome advancement would be machines that have the capacity to make intuitive decisions independently, as this would ease the decision making process. For example, in education, there is a lot of talk of the possibilities of ECG sensors for monitoring the assimilation capacity of students. However, it is one thing to point out that students are not assimilating correctly, and it is another to identify why there is a lag in concentration and what can be done to rectify this. While this example may seem like a controlled society almost like the fictional ‘big brother’, it would still be pretty impressive to see machines that are not only capable of gathering information but acting upon it.
– Lack of theory
Another big problem that deep learning faces is that there is an astounding lack of theory that surrounds the creation of models. For example, there is the possibilities of technology in health sciences to assimilate information concerning the lifestyle of an individual, then supply this information to a model or algorithm from which useful decisions can be made. However, there is no sound theory behind the models that are derived. The only models that can be fed with this information are the ones that are generated by a human imprint. This is a big problem when it comes to machine learning, and it still points back to the inability of the machines to function independently of a human instead of being able to come to reasonable deductions on its own. This tends to create a ‘black hole’ feeling for this technology, because there is just so much information but so little to do with it.
– The problem of unusual events
Machine learning thrives on the ability of machines to assimilate information and made predictions based on the data generated. However, this becomes a problem when there is the occurrence of an unusual events. The computers would find it hard to make predictions when there is the possibility of an unusual event. This is often called a ‘zero day’ event, and there is no way of identifying the reason for its unusual occurrence. For example, there is the possibility of predicting a trend in the lifestyle of people diagnosed with cancer, but there is always that anomaly. This unusual occurrence makes it very hard for machine learning to perform optimally. It is therefore an impossibility of machine learning to predict the occurrence of these unusual events.
– Incorrect data
Machine learning is also very terrible at dealing with information that is not correct. It can also not make any corrections on this without human interference. For example, if the time on a particular device is off by about a minute, it associates timestamps in all the respective log files with that error. This throws a monkey wrench into the analysis.
Brought to you by the RobustTechHouse team. If you like our articles, please also check out our Facebook page.
Also published on Medium.
Great article! Thank you for sharing attractive and impressive article.
Elevate your career prospects with our groundbreaking Citrix exam online access for 2023. Seamlessly designed for the digital age, this innovative platform redefines convenience and flexibility. Now, you can prepare and certify from anywhere, at your own pace. Experience immersive learning, real-world scenarios, and expert guidance that ensures you’re Citrix-ready. With up-to-date content and interactive simulations, success is within reach.
Don’t miss this opportunity to stay ahead in the ever-evolving tech landscape. Unlock your potential and excel in the world of Citrix. Embrace the future, today!