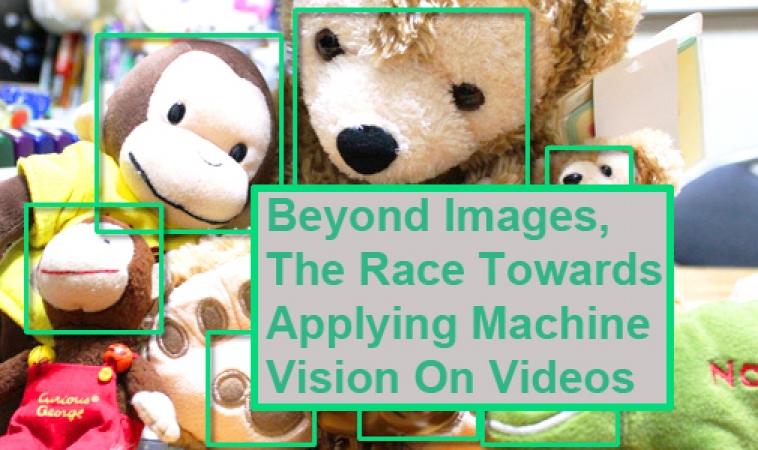
Beyond Images, The Race Towards Applying Machine Vision On Videos
“Beyond images, the race towards applying machine vision on videos” Are we there yet when it comes to applying visual recognition on videos? What are the challenges still and what are we going to expect beyond videos?
Taking it further, machine vision with the help of image capturing analysis automates many tasks at the commercial level which include counting, inspection, gauging and reading optical characters and barcodes. Usually, human effort is required to undertake the inspection challenges, however with the help of advanced hardware and software systems used in machine vision, it is easier to perform the above mentioned functions with more precision, reliability and at higher speeds.
When we talk about applying machine vision on videos, it is essential for us to discuss visual recognition and object recognition in videos.
The Visual Recognition service helps to analyze the visual appearance of video frames or images to comprehend the action in a particular scene. Semantic classifiers with the help of machine learning technology recognize several visual entities such as settings, objects, and events.
Numerous multinational companies like Unilever, Vodafone and Trivago are widely using the concept of visual recognition in their videos and why not, after all it is not only about having a pair of eyes in terms of camera; it is about having a brain with it as well.
Developments in Deep Learning and concept specialization from companies like “Clarifai” and “Dextro” has resulted in notable achievements in this field. “Clarifai’s” powerful image and video recognition technology is built on the most advanced machine learning systems and made easily accessible by a clean API, empowering developers all over the world to build a new generation of intelligent applications.
The companies mentioned above and many others are progressing day by day in order to develop flawless visual recognition engines having unparalleled performance. The basic goals are to achieve:
- Accuracy: The aim is to develop such visual recognition systems that can surpass the human accuracy in recognizing the tasks.
- Performance Oriented: Ensuring that the feature processing time is much faster than the human annotators. For this, certain algorithms are developed to classify the images in microseconds.
- Scalability: Every user and business has their own demand. Visual recognition software are built upon the model of scalability to ensure that countless requests are met every day.
Every innovation is becoming history with each passing day. All thanks to this era of record breaking transformation. Every technology ends up being greeted by its successor in literally no time at all. However, we can see visual recognition still going through that phase of development with an aim to achieve mastery. But at the same time, the present developments have been remarkable, especially “automatic extraction of evidence / desired results from a certain footage”.
Saving administration and operational costs along with the least amount of time invested in order to categorize certain evidences and results has been a great achievement in this field. This has resulted in search and retrieval from databases in a much more accurate, flexible and secure manner.
Machine vision is not just about visual recognitions and videos. The way technologies have synchronized, and with the developments in various industries taking place, it offers machine vision a huge platform to expand. Extensions and developments in the field of Computer vision have helped transformation and refinement of Machine vision which is considered an integral part of it while focusing on industrial applications.
Despite the quality of this research and considerable developments in machine vision and machine learning techniques, it is difficult to replace certain tasks that involve classification or selection of a certain feature.
Machine vision is on its way and has already made remarkable discoveries, however the challenges are still present.
Let alone, object/visual recognition, many interesting problems in machine vision are yet to be discussed upon and to be researched in order to build a deep understanding to initiate relevant developments . These include more difficult tasks such as joint geometric / semantic scene parsing, modeling and redefining of visual attributes, object discovery. Image aesthetics, etc.
Talking more about the challenges faced, even within the field of “classic” recognition systems, significant challenges are faced in scaling up machine learning techniques and the concept and importance of machine vision to millions of images and thousands of categories (for example the ImageNet data set).
Developments in the field of unsupervised learning can bring a lot of improvements and can help in eliminating the current and future challenges.
Brought to you by the RobustTechHouse team (A top app development company in Singapore). If you like our articles, please also check out our Facebook page.