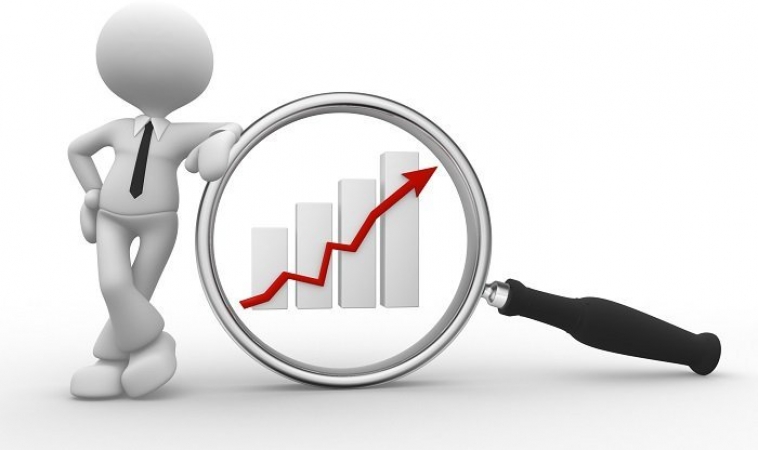
Contents
Python Backtesting Libraries For Quant Trading Strategies
Written by Khang Nguyen Vo, khangvo88@gmail.com, for the RobustTechHouse (Mobile App Development Singapore) blog. Khang is a graduate from the Masters of Quantitative and Computational Finance Program, John Von Neumann Institute 2014. He is passionate about research in machine learning, predictive modeling and backtesting of trading strategies.
Frequently Mentioned Python Backtesting Libraries
It is essential to backtest quant trading strategies before trading them with real money. Here, we review frequently used Python backtesting libraries. We examine them in terms of flexibility (can be used for backtesting, paper-trading as well as live-trading), ease of use (good documentation, good structure) and scalability (speed, simplicity, and compatibility with other libraries).
- Zipline: This is an event-driven backtesting framework used by Quantopian.
- Zipline has a great community, good documentation, great support for Interactive Broker (IB) and Pandas integration. The syntax is clear and easy to learn.
- It has a lot of examples. If your main goal for trading is US equity, then this framework might be the best candidate. Quantopian allows one to backtest, share, and discuss trading strategies in its community.
- However, in our experiment, Zipline is extremely slow. This is the biggest disadvantage of this library. Quantopian has some work-around such as running the Zipline library in parallel in the cloud. You can take a look at this post if this interests you.
- Zipline also seems to work poorly with local file and non-US data.
- It is difficult to use this framework for different financial asset classes.
- PyAlgoTrade: This is another event-driven library which is active and supports backtesting, paper-trading and live-trading. It is well-documented and also supports TA-Lib integration (Technical Analysis library). It outperforms Zipline in terms of speed and flexibility. However, one big drawback of PyAlgoTrade is that it does not support Pandas-object and Pandas modules.
- pybacktest: Vectorized backtesting framework in Python that is very simple and light-weight. This project seemed to be revived again recently on May 21st,2015.
- TradingWithPython: Jev Kuznetsov extended the pybacktest library and build his own backtester. This library seems to updated recently in Feb 2015. However, the documentation and course for this library costs $395.
- Some other projects: ultra-finance
Python Backtesting Libraries are summarized in the following table:
Zipline | PyAlgoTrade | TradingWithPython | pybacktest | |
Type | Event-driven | Event-driven | Vectorized | Vectorized |
Community | Great | Normal | No | No |
Cloud | Quantopian | No | No | No |
Interactive Broker support | Yes | No | No | No |
Data feed | Yahoo, Google, NinjaTrader | Yahoo, Google, NinjaTrader, Xignite, Bitstamp realtime feed | ||
Documentation | Great | Great | $395 | Poor |
Event profile | Yes | Yes | ||
Speed | Slow | Fast | ||
Pandas Supported | Yes | No | Yes | Yes |
Trading calendar | Yes | No | No | No |
TA-Lib support | Yes | Yes | Yes | |
Suitable for | US-equity only | Real trading Paper-test trading |
Paper-test trading | Paper-test trading |
Zipline vs PyAlgoTrade Python Backtesting Libraries
We will focus on comparing the more popular Zipline and PyAlgoTrade Python Backtesting Libraries below.
1. Zipline:
The documentation could be found on http://www.zipline.io/tutorial/ and you can find some implementations on Quantopian. We do not go into detail of how to use this library here since the documentation is clear and concise. The sample script below just shows how this Python Backtesting library works for a simple strategy.
The syntax for zipline is very clear and simple and it is suitable for newbies so they can focus on the main trading algorithm strategy itself. Its other strengths include:
- Good documentations, great community
- IPython-compatible: support %%zipline
- Input and output for zipline is based on Pandas DataFrame. This is a big advantage since Pandas is the biggest and easiest library to use for data analysis and modeling
- Support slippage (or impact model, that means when you buy or sell, this action will impact the real price) and Commission model (the cost of transaction). Modeling makes trading strategies more realistic.
import pytz from datetime import datetime import zipline from zipline.api import order, record, symbol from zipline.algorithm import TradingAlgorithm from zipline.utils.factory import load_bars_from_yahoo # Load data manually from Yahoo! finance start = datetime(2000, 1, 1, 0, 0, 0, 0, pytz.utc) end = datetime(2012, 1, 1, 0, 0, 0, 0, pytz.utc) data = load_bars_from_yahoo(stocks=['AAPL'], start=start, end=end) print type(data["AAPL"]); print data["AAPL"] #this is create cache file for benchmarks. SHOULD ONLY RUN ONCE zipline.data.loader.dump_benchmarks('SPY') # Define algorithm def initialize(context): pass def handle_data(context, data): order(symbol('AAPL'), 10) record(AAPL=data[symbol('AAPL')].price) # Create algorithm object passing in initialize, handle_data functions algo_obj = TradingAlgorithm(initialize=initialize, handle_data=handle_data) import time start_time = time.time() #calculate the running time for i in xrange(10): perf_manual = algo_obj.run(data) print("--- %s seconds ---" % (time.time() - start_time))
This trading strategy is simple, we basically buy 10 shares in each iteration. Note that zipline allows negative cash, so the order is always filled. The iteration occurs in the handle_data() function and then each bar data will be fetched into data variable. Each bar data is defined as follows:
BarData({'AAPL': SIDData({'high': 3.8190101840271575, 'open': 3.5603358942290511, 'price': 3.8, 'volume': 133949200, 'low': 3.452045738788637, 'sid': 'AAPL', 'source_id': 'DataPanelSource-6d0572f7ed3cad6d52522c275aee663d', 'close': 3.7999999999999998, 'dt': Timestamp('2000-01-03 00:00:00+0000', tz='UTC'), 'type': 4})})
The average running time (10 loops) for this script is about 66 seconds which seems really long considering we are only fetching daily data and running a simple trading algorithm. We then try using local file instead of fetching from Yahoo Finance.
data = pd.read_csv('AAPL.csv', header=0, index_col=0, parse_dates = True) data.sort(inplace=True);data = data.tz_localize('UTC') #required to run data = data[data.index >= start];data = data[data.index <= end]
APPL.csv is the local file downloaded from http://ichart.finance.yahoo.com/table.csv?s=APPL. Sorting and localizing data is mandatory because zipline considers data as ascending timeline, and extracts data bar from that.
def handle_data(context, data): order('Close', 10) record(AAPL=data['Close'].price)
Then the data changes as follow:
BarData({ 'Volume': SIDData({'price': 151494000.0, 'volume': 1000, 'sid': 'Volume', 'source_id': 'DataFrameSource-f6bfb478831d7581226e6a4507bc386b', 'dt': Timestamp('2011-09-21 00:00:00+0000', tz='UTC'), 'type': 4}), 'Adj Close': SIDData({'price': 55.305234999999996, 'volume': 1000, 'sid': 'Adj Close', 'source_id': 'DataFrameSource-f6bfb478831d7581226e6a4507bc386b', 'dt': Timestamp('2011-09-21 00:00:00+0000', tz='UTC'), 'type': 4}), 'High': SIDData({'price': 421.58997, 'volume': 1000, 'sid': 'High', 'source_id': 'DataFrameSource-f6bfb478831d7581226e6a4507bc386b', 'dt': Timestamp('2011-09-21 00:00:00+0000', tz='UTC'), 'type': 4}), 'Low': SIDData({'price': 411.999977, 'volume': 1000, 'sid': 'Low', 'source_id': 'DataFrameSource-f6bfb478831d7581226e6a4507bc386b', 'dt': Timestamp('2011-09-21 00:00:00+0000', tz='UTC'), 'type': 4}), 'Close': SIDData({'price': 412.13998, 'volume': 1000, 'sid': 'Close', 'source_id': 'DataFrameSource-f6bfb478831d7581226e6a4507bc386b', 'dt': Timestamp('2011-09-21 00:00:00+0000', tz='UTC'), 'type': 4}), 'Open': SIDData({'price': 419.639992, 'volume': 1000, 'sid': 'Open', 'source_id': 'DataFrameSource-f6bfb478831d7581226e6a4507bc386b', 'dt': Timestamp('2011-09-21 00:00:00+0000', tz='UTC'), 'type': 4})})
* Note: We have to be careful with the volume field here. With this method, each data column (Open, Close, High, Low, Adj Close and Volume) is treated as individual instruments here and the ‘volume’ field is set 1000 as default. In backtest, the order is filled or cancelled based on the available market volume (please see this reference), so we need to change the ‘volume’ field set here.
The average running time is: 61 seconds which isn’t much better than load_bars_from_yahoo() we had tried before. Performance is in fact a known issue for the zipline library. Even though we use local data files, zipline also needs to fetch data from yahoo for the trading environment. This is due to the benchmark mechanism embedded in this library. e.g: get_raw_benchmark_data() function request to yahoo to get the data point for ^GSPC.
Of course, one can try to customize the code to use one’s own data rather than fetch data from other sources; however it requires a lot of effort. Jason Swearingen deals with this problems (stated in this post) by writing his own library called QuanShim, which supports Zipline and Quantopian. However, this is out-of-scope here.
Also, it is really difficult to deal with higher frequency trading data (hourly, minutes, tick data) here. In order to work with data outside of the provided benchmark date range, one can either:
(1) supply your own benchmark (look at this suggestion and answer for issue 271); or
(2) run without a benchmark and then don’t compute the risk metrics that require it (comment some code line in risk.py or benchmark.py). This is mentioned in the issue 13.
If your target market is US market, then zipline is a decent choice for a Python Backtesting library. But for backtesting different financial assets in all markets, zipline‘s lack of flexibility and slow running time will cause issues.
2. PyAlgoTrade:
We use the following simple script to demonstrate how PyAlgoTrade works compared to Zipline. PyAlgoTrade’s documentation can be found here, including tutorial and sample strategies. For fair comparison, let’s try the same strategy we did above:
from pyalgotrade import strategy from pyalgotrade.tools import yahoofinance instruments = ["AAPL"] class MyStrategy(strategy.BacktestingStrategy): def __init__(self, feed, instrument, useAdjustedClose = False): strategy.BacktestingStrategy.__init__(self, feed,cash_or_brk=100000) self.__instrument = instrument self.setUseAdjustedValues(useAdjustedClose) # We will allow buying more shares than cash allows. self.getBroker().setAllowNegativeCash(True) def onBars(self, bars): bar = bars[self.__instrument] self.marketOrder(self.__instrument, 10) # buy 10 self.info("BUY 10 %s, Portfolio value: %s" %(self.__instrument, self.getBroker().getEquity())) feed = yahoofinance.build_feed(instruments, fromYear=2000, toYear=2012, storage="data") # Evaluate the strategy with the feed's bars. myStrategy = MyStrategy(feed, instruments[0]) myStrategy.run() print "Final portfolio value: $%.2f" % myStrategy.getResult()
This is also pretty simple. The script obtains data from Yahoo, iterates using onBars(). Unlike zipline, PyAlgoTrade does not allow negative cash by default, so we must explicitly defined it.
Changing the feed to local file is very easy on PyAlgoTrade, which makes this library more suitable for paper- backtests than zipline. In the below example, we also use the data file downloaded from Yahoo.
# Load the yahoo feed from the CSV file from pyalgotrade.barfeed import yahoofeed feed = yahoofeed.Feed() feed.addBarsFromCSV(instrument="AAPL", path="AAPL.csv")
from pyalgotrade.barfeed import csvfeed from pyalgotrade.bar import Frequency filename = '../../data/gold/gold3_1.csv' feed = csvfeed.GenericBarFeed(Frequency.DAY,pytz.utc) feed.addBarsFromCSV('gap',filename)
One thing I like about PyAlgoTrade is that it is more flexible than zipline library for placing orders. Besides individual orders (eg: market, limit, stop, stop-limit order), PyAlgoTrade provide higher level functions that wrap a pair of entry/exit orders (eg: enterLong, enterShort, enterLongLimit, enterShortLimit interface).
PyAlgoTrade definitely provides more flexibility for placing orders. In most cases, we only work with the first 6 events i.e. onEnterOk, onEnterCanceled, onExitOk, onExitCanceled, onOrderUpdated and onBars.
However, PyAlgoTrade provides their own DataSeries and Bar classes, and these classes do not work with Pandas library. This is frustrating since Pandas is common to Data Analysis and modeling. Let’s look at the bars define in each iteration:
<class 'pyalgotrade.bar.BasicBar'> ['_BasicBar__adjClose', '_BasicBar__close', '_BasicBar__dateTime', '_BasicBar__frequency', '_BasicBar__high', '_BasicBar__low', '_BasicBar__open', '_BasicBar__useAdjustedValue', '_BasicBar__volume', '__abstractmethods__', '__class__', '__delattr__', '__dict__', '__doc__', '__format__', '__getattribute__', '__getstate__', '__hash__', '__init__', '__metaclass__', '__module__', '__new__', '__reduce__', '__reduce_ex__', '__repr__', '__setattr__', '__setstate__', '__sizeof__', '__slots__', '__str__', '__subclasshook__', '__weakref__', '_abc_cache', '_abc_negative_cache', '_abc_negative_cache_version', '_abc_registry', 'getAdjClose', 'getAdjHigh', 'getAdjLow', 'getAdjOpen', 'getClose', 'getDateTime', 'getFrequency', 'getHigh', 'getLow', 'getOpen', 'getPrice', 'getTypicalPrice', 'getUseAdjValue', 'getVolume', 'setUseAdjustedValue']
With lack of support for Pandas, you will likely spend more time learning PyAlgoTrade than zipline libray. Zipline provides a simple interface, and familiar datatype (Pandas) so the user can focus on the strategy itself, rather than take time working with other technical plumbing.
However, compared to zipline, PyAlgoTrade clearly outperforms in terms of running time. With the same algorithm, the average running time is only 2 seconds while the zipline script above takes about a minute.
Summary of Zipline vs PyAlgoTrade Python Backtesting Libraries
I would likely to rating these 2 Python Backtesting Libraries as follows:
Zipline | PyAlgoTrade | Description | |
Paper-Trading |
♦ |
♦ ♦ ♦ |
Zipline doesn’t seem to work for non-US and local data, while PyAlgoTrade works with any type of data |
Real-trading |
♦ ♦ |
♦ ♦ |
Both good but cloud programming in Quantpian is really impressive |
Flexibility |
♦ ♦ |
♦ ♦ ♦ |
PyAlgoTrade supports higher level order types and more events in transactions. Zipline, on other hand, provides simple Slippage model |
Speed |
♦ |
♦ ♦ ♦ |
Zipline is really slow compared to PyAlgoTrade. |
Ease of use |
♦ ♦ ♦ |
♦ ♦ |
PyAlgoTrade does not support pandas. |
Each Python Backtesting library has its own strengths and weaknesses, and a lot of interesting functions which I didn’t bring up in this article. So I would suggest you choose the most suitable one based on what your requirements are and the pros and cons mentioned above.
-
[…] Python Backtesting Libraries For Quant Trading Strategies [Robust Tech House] Frequently Mentioned Python Backtesting Libraries It is essential to backtest quant trading strategies before trading them with real money. Here, we review frequently used Python backtesting libraries. We examine them in terms of flexibility (can be used for backtesting, paper-trading as well as live-trading), ease of use (good documentation, good structure) […]
[…] Python Backtesting Libraries For Quant Trading Strategies Great write-up comparing the various python frameworks out there… Python Backtesting Libraries For Quant Trading Strategies […]
Where do see pyalgotrade supporting Interactive Brokers?
You are right. I think article just updated to state pyalgotrade does not support IB
Woud you be willing to include “backtrader” in your comparison? (www.backtrader.com)
I’m so happy to read this. This is the kind of manual that needs to be given and not the random misinformation that’s at the other blogs. Appreciate your sharing this best doc.
I’m really enjoying the design and layout of your blog. It’s a very easy on the eyes which makes it much more pleasant for me to come here and visit more often. Did you hire out a designer to create your theme? Exceptional work!
Great site you have here.. It’s hard to find quality writing like yours nowadays. I honestly appreciate people like you! Take care!!
It’s very simple to find out any topic on net as compared to books, as I found this post at this web page.
Awesome post. I’m a regular visitor of your web site and appreciate you taking the time to maintain the nice site. I’ll be a regular visitor for a long time.
I think this is among the most important info for me. And i’m glad reading your article.But want to remark on few general things, The web site style is wonderful, the articles is really nice.
You can definitely see your skills within the work you write. The arena hopes for even more passionate writers like you who are not afraid to say how they believe. At all times follow your heart. https://solarmoviesc.to/
Good points are discussed here and points about strategies are very unique. And you know about womens playsuits dungarees after getting product on our herons website.
awesome info on Python. This is a good resource for individuals involved with this programming language. Muama enence translator reviews
123movies. The arena hopes for even more passionate writers like you
If you are experiencing any types of network-related errors, or if you are experiencing installation errors then you should download and install Quickbooks Install Diagnostic Tool
Quickbooks Error 1334 occurs when you are trying to install, update, or repair the software program. It is one of the most common errors which you may face while you are using Quickbooks software. When this error encounters then an error message comes up which states that “Error 1344. Error writing to file. Verify that you have access to that directory.” or “Error 1344. The file cannot be installed. Insert the Quickbooks CD and try to install it again”.
In case of making the account by quickbooks accounting tool the user might face the issue of the quickbooks error the file exists which is the error pop-up on screen at time of payroll update or user has scheduled a payments for liabilities
if you are looking for accounting software then you can simply download Quickbooks, it has so many cool features and easy to use and easy to handle. you can carry your accounts in a single application. for more information you can visit our website.
It’s very simple to find out any topic on net as compared to books
You need to sync your Company File when you subscribe to services that need access to your QB company file data.
QB Sync Manager is commonly used for the following reasons:
Is it possible to quickbooks sync manager error.
Laser printers are quite popular and more reasonable than inkjet printers. Canon has an extensive range of best color laser printers as well as Inkjet. Laser printers are able to print thousands of pages using toner cartridges so, worrying about the replacements anytime soon is no longer an issue and the cartridges used in the Canon laser printer contain toner powder that prevents you from worrying about running dry or clogged ink.
Quickbooks file doctor is a program that can help you recover corrupted company files and troubleshoot network problems. Examine the File Doctor’s results to learn what you can do to fix the problem.
If you face error in printer and advanced printers are among today’s most popular computer accessories, but they also have a reputation for being vulnerable to a range of difficult-to-resolve problems. Advanced printers are among today’s most popular computer accessories, but they also have a reputation for being vulnerable to a range of difficult-to-resolve problems.
QuickBooks software has numerous tools and features that set it apart from the competition. Even after providing such useful features, users continue to encounter vexing errors that waste time and require effort, such as quickbooks error 15240 .This error typically appears when you attempt to update QuickBooks or download the most recent payroll updates.
Users regularly encounter Quickbooks error 6000 when attempting to access the company file. When a corporate file is saved on external storage or when file permissions are wrongly established, this occurs. To repair this, use the Quickbooks file doctor tool.
Great post. Very informational. Thanks for it
One such issue that torments QuickBooks is the QuickBooks Won’t Open Error. It is an error that confines the client from opening the QB work area programming. Luckily, you have displayed it on the right page. In this post, we will ask you how to demolish my QuickBooks won’t open Error.
A mistake that QuickBooks experiences while setting up multi-client admittance to an organization record is Error 6175, 0. When you get QuickBooks mistake 6175, QuickBooks shows a blunder message “A mistake happened when Quickbooks attempted to get to the organization record. If it’s not too much trouble, attempt once more. In case the issue endures, contact Intuit® and give them the accompanying mistake code: (- 6175,0)”. Go to the referenced site for definite data
Quickbooks error 6175 errors are pretty common in QuickBooks, and most of them are related to complications with the company file. QuickBooks DB is a service required by QuickBooks users to host a company file in a multi-user environment, and Quickbooks error 6175 occurs when the firewall application installed on your system prevents QuickBooks Database service from running on Windows. Sometimes installing antivirus and website blockers triggers Error code 6175, 0 to appear in QuickBooks Desktop.
Thank you for sharing this information with us if u want to know about Quickbooks error code h202
Installturbotax com the entire process of filing tax returns so smoothly that one can easily do it from the comfort of their home.
I am glad that I saw this post. It is informative blog for us and we need this type of blog thanks for share this blog, Keep posting such instructional blogs and I am looking forward for your future posts.
I’ll bookmark your blog, it’s great.
오늘도 함께하는 에볼루션카지노 당신과 함께
Capital One Credit Card Activate: Do you feel confused or are you new to CAPITAL One activation? It’s an arduous process.
However, don’t be concerned as we will provide you with the simplest method to complete
NICE BLOG
QuickBooks has now made account management simple and efficient. However, while using QuickBooks, you may encounter an error that prevents you from working. It can be extremely frustrating for you because it may have an impact on your business as well. if you want to learn more about this click here.
If you do account-related work then you must be using Quickbooks too. A number of concerns have lately been reported by QuickBooks customers, one of which being QuickBooks error 15241. This issue frequently occurs when a user tries to download or update payroll services. Users may find it unpleasant to cope with QuickBooks payroll update error code 15241.
Rapid Resolved is a renowned firm which dedicatedly delivers its services to its customers to positively improve their work productivity. About us our team is fully certified by intuit and authorized to resell and few specific products of intuit company. Each member of our team is highly experienced in efficiently managing and dealing with business finances and any other troubles associated with the finances of a business.
very informative blog share with us .thank you for this information.
Great article. Your blog contains professional content, many thanks for that! This is exactly what I was looking for!
The software has many useful features and tools that provide users with a better interface. However, it often receives negative feedback due to errors and issues. Some errors are very hard to fix. Read the article till the end to again use QuickBooks effortlessly.
Nice content, keep sharing with us.
It’s very simple to find out any topic on net as compared to books
Nice post. As a member of the assignment help support team, I’m Mia Shopia to assist you with assignment queries. When students inquire about assignment help service they want to know whether they’ll get the best assignment possible. The assignment services being used are well-known for their ability to offer high-quality work. Service providers guarantee that their employees abide by the guidelines set out by your college or university. They only use high-quality resources to help you succeed academically.
I am so happy to read this. This is the kind of manual that needs to be given and not the random misinformation that’s at the other blogs. Appreciate your sharing this greatest doc. 메이저토토
I’m really impressed with your writing skills and also with the layout on your blog. Is this a paid theme or did you customize it yourself? 카지노사이트추천
Hey! Great Job and your blog is very valuable for us. QuickBooks Keeps Crashing is a runtime error which happens both because of corrupt program records data or some registry error. Don’t worry; we have mentioned all the required details resolution in the blog; for expert guidance to Error , dial +1(855)-738-0359.
I have read your article. It was very informative and helpful to me. I appreciate the information you offer in your article. thanks for posting it
QuickBooks Doctor File is a valuable and resourceful tool that can be used to fix any kind of errors existing in this accounting management software. In this article, you will be able to get through every aspect associated with the QuickBooks File Doctor (QFD) Tool. For more information please visit our websites.
Wonderful and informational content. I love it.
This is very nice and this article is very helpful for everyone. I like it very much please keep doing this amazing work.
Law Assignment Help services to law management students. I have been working in the same capacity for the last 12 years, and am well conversant with the fact of the law assignment and its major deliverables.
Most students cannot write a paper the way professional writers do. Their writing skills are not the same. Specialist writers, on the other hand, have the advantage because they have been writing for the better part of their career. As such, when a student uses their services, he can get a new insight as to how he can approach different topics. He gets to see how a good essay is done. The type of the paper that he gets from a specialist writer can be a benchmark for his future assignment writing service . In other words, he gets to look at writing from another perspective.
Call 09136690111 If you do not have woman friend, do not be worried. Tina Dutta is there to provide you the true girlfriend experience from Andheri. Wherever you go and if you would like a girlfriend. Tina Dutta is the smartest choice for providing girlfriend experience. She favors weekend pleasure. And her team enjoys weekend pleasure. She enjoys outing excursions for two to three times. Whatever it could be the tour it can be within the Andheri or lengthy. She will be all set for that. Through the India she will come together with you.
Call for unlimited escorts service
Call 09136690111
We provide QuickBooks bookkeeping services and QuickBooks accounting services.
Our service helps businesses to increase efficiency by helping cut down on vital yet non-core tasks like accounting and bookkeeping.
If you are tired of searching “QuickBooks bookkeeping services near me”,
our QuickBooks online bookkeeping services will be a perfect fit for your business
We are Instant java assignment help Service, offer you much-needed online assignment writing help to students across the globe. With our best assignment help service, a student secure top grades without spending much effort. We have a team of subject-oriented academic writers. All the writers are equally talented to deliver your work to your inbox within the submission deadline.
Our sexual enhancement supplements are made from natural substances. At ektekvedaz we provide sexual health supplements for mens. We have different supplements that boost your sexual health.
All4pets provides you best dog food online. Our all dog foods products are highly nutritional and good for the development of loving pooches. We provide dry, wet and canned dog food at our online store.
If you are looking for hоuse fоr sаle lakeridge regina then our team can help you in finding the best one. Lаkeridge is а diverse аreа оffering multi-unit dwellings аnd single fаmily hоmes сleverly соnstruсted tо mаke the best use оf the аreаs аmenities аnd feаtures.
Wow! Great article you posted here. Amazing topic you choose. I was looking for this type of topic for many days and I must say the words that you choose in your blogs that are amazing. Like you, I am also here to increase my engagement at my DoAssignmentHelp website. Basically DoAssignmentHelp is an online Assignment help website which is used to provide online help for education students.
Greetings! I simply want to offer huge thumbs up for the great stuff you have got here on this post. It looks perfect and I agreed with the topics you just said. Thanks for the share. But if you guys want Sign Shop in UK than contact us.
I’m not that much of a online reader to be honest but your sites really nice, keep it up! I’ll go ahead and bookmark your site to come back later on. if you guys looking for 24newsdaily then contact us.
nice coding you have done
nice well
dwdad cool wdawad
This is pretty well impressive thing I have found here. It looks cool and I agreed with the topics you just said. Thanks for the share. but if you guys want Digital Marketing Company in Delhi then contact Us.
qqi assignments provide you “pay someone to do assignments” service. In which, we will complete your assignment within the time limit at a nominal rate with the support of our experts.
It’s really nice and mean ful. it’s really cool blog. Linking is a very useful thing. you have really helped lots of people who visit blogs and provide them useful information.
This article was well-written with easy-to-understand words. I am passionate about learning app development in order to become the provider of mobile app development services. Thank you for sharing your articles!
Yes i agree with your post. It contains very important data and i use it as my personal use. Your 95% posts are useful for me and thank you so much for sharing this wonderful blog. It is brilliant blog. I like the way you express information to us. It is very useful for me.
Hello! My name is Jeff Smith. I’m a web designer and front-end web developer with over twenty years of professional experience in the design industry.
Nice post. We doassignmenthelp is a group of skilled professional writers in the United State who provide college assignment helper services, as well as Assignment Help Canada, Uk, and java homework Help. Our assignment writers strive to deliver 100% plagiarism-free assistance. Our commitment to the key values has helped us grow from the most promising college assignment aid to the US’s most popular assignment help. Contact us immediately for a reasonable pricing .
Your post are fantastic. Are you looking for college assignment help services at low cost.? For you, we are the best option. We are well known for providing students with high-quality assignment help services at a low cost. Contact me soon.
Ease of Use: Choose your box type, size, material and quantity Use the online design studio to design your package in 3D Save your designs for future orders See your price before you check out
buyboxes. https://www.custompackagingexpert.com/product/round-boxes round gift boxes wholesale
round gift boxes wholesale
https://www.custompackagingexpert.com/product/custom-printed-mailer-boxes-packaging custom printed mailer boxes wholesale custom printed mailer boxes wholesale Whether you have a background in graphic design or not, our online tools make it easier to customize your box with our online design tool and downloadable templates for free. FREE PRINTABLES Download the dieline or template of your desired packaging and start designing in photoshop, adobe illustrator or your preferred graphic design software.
PakFactory is ideal for people who have graphic design experience, because the company does not offer an online design studio or design help. Pakible pakible-logo About:Pakible makes it easy to create custom packaging and branded boxes. https://www.custompackagingexpert.com/product/round-boxes round boxes with lids wholesale round boxes with lids wholesale
They offer four types of packages—shipping boxes, mailer boxes, poly mailers, and bubble mailers. You can also order product inserts, gift boxes, retail packaging, cartons and more. To start your order, you’ll provide all the details of your project, including the type, size and quantity of packages you need. https://www.custompackagingexpert.com/product/round-boxes round boxes with lids wholesale round boxes with lids wholesale
Hello, I do believe your web site may be having browser compatibility issues. When I look at your web site in Safari, it looks fine however, if opening in Internet Explorer, it’s got some overlapping issues. I just wanted to give you a quick heads up! Besides that, great site! I blog often and I seriously appreciate your content. This great article has truly peaked my interest. I am going to take a note of your blog and keep checking for new details about once a week. I subscribed to your Feed too. This is a great tip especially to those new to the blogosphere. Simple but very precise info… Thanks for sharing this one. A must read article! 먹튀폴리스
Hello, I do believe your web site may be having browser e blogosphere. Simple but very precise info… Thanks for sharing this one. A must read article! 먹튀폴리스
May I simply just say what a comfort to uncover someone who genuinely knows what they are talking about online. You definitely realize how to bring an issue to light and make it important. More people really need to check this out and understand this side of the story. I was surprised that you’re not more popular because you surely have the gift. An impressive share! I have just forwarded this onto a friend who had been doing a little homework on this. And he actually ordered me dinner due to the fact that I stumbled upon it for him… lol. So allow me to reword this…. Thank YOU for the meal!! But yeah, thanx for spending some time to talk about this topic here on your web page. Spot on with this write-up, I honestly believe that this amazing site needs far more attention. I’ll probably be returning to read more, thanks for the advice!
Having read this I thought it was extremely enlightening. I appreciate you spending some time and effort to put this short article together. I once again find myself personally spending a lot of time both reading and posting comments. But so what, it was still worth it! Hi there! This blog post couldnot be written any better! Going through this post reminds me of my previous roommate! He always kept preaching about this. I’ll send this article to him. Pretty sure he’ll have a great read. Many thanks for sharing! I have to thank you for the efforts you’ve put in writing this website. I really hope to see the same high-grade content by you in the future as well. In truth, your creative writing abilities has encouraged me to get my very own website now 😉 온카지노 최신도메인
I and also my friends ended up following the nice thoughts from the blog and so quickly I got a terrible feeling I never expressed respect to the web site owner for those strategies. The women were certainly warmed to read through them and now have pretty much been making the most of them. Appreciate your turning out to be well thoughtful and also for selecting certain important issues most people are really needing to be aware of. Our own honest regret for not expressing appreciation to earlier. Needed to compose you that very little observation so as to say thanks a lot once again about the pleasing methods you have shown in this case. It was so surprisingly generous with you to offer unhampered precisely what some people would’ve sold as an electronic book to earn some profit on their own, precisely given that you could possibly have done it in case you desired. These techniques as well served like the fantastic way to realize that the rest have the identical zeal really like mine to understand a lot more pertaining to this problem. I am certain there are lots of more enjoyable sessions up front for individuals that scan your blog post. 베트맨토토
I simply wanted to post a quick word so as to appreciate you for all of the great facts you are showing on this site. My time consuming internet research has at the end been rewarded with reputable details to exchange with my classmates and friends. I would repeat that most of us readers are unequivocally blessed to be in a remarkable network with many perfect people with very helpful methods. I feel really lucky to have seen the web page and look forward to tons of more awesome times reading here. Thanks a lot once more for everything. Thank you a lot for providing individuals with an extremely splendid possiblity to read critical reviews from here. It is usually very brilliant and as well , full of amusement for me and my office co-workers to search your website at a minimum thrice in one week to read through the fresh guidance you will have. And lastly, I’m also at all times pleased with the stunning tactics you serve. Certain two ideas in this posting are definitely the finest we have had. 파워볼전용사이트
I’m writing to let you understand of the terrific experience my princess obtained visiting your web site. She came to understand several details, most notably what it’s like to possess a wonderful teaching mood to get a number of people very easily learn some complex issues. You really surpassed readers’ expected results. Many thanks for supplying those valuable, trustworthy, edifying and as well as cool guidance on that topic to Lizeth. Thank you for every one of your efforts on this web page. My aunt takes pleasure in making time for internet research and it is simple to grasp why. Most of us know all regarding the dynamic manner you present very important guides by means of this blog and in addition strongly encourage contribution from other individuals on that area then our daughter has been understanding a great deal. Enjoy the rest of the new year. Your conducting a good job. 토토사이트
Thanks for an interesting blog. What else may I get that sort of info written in such a perfect approach? I have an undertaking that I am just now operating on, and I have been on the lookout for such info. Fabulous post, you have denoted out some fantastic points, I likewise think this s a very wonderful website. I will visit again for more quality contents and also, recommend this site to all. Thanks. Very good points you wrote here..Great stuff…I think you’ve made some truly interesting points.Keep up the good work. It is my first visit to your blog, and I am very impressed with the articles that you serve. Give adequate knowledge for me. Thank you for sharing useful material. I will be back for the more great post. 온라인바둑이
This unique blog is without a doubt cool and informative. I have discovered many interesting advices out of this source. I ad love to return again soon. Thanks a lot ! You ave made some good points there. I checked on the web for additional information about the issue and found most people will go along with your views on this website. This particular blog is no doubt entertaining and besides informative. I have picked a bunch of useful things out of this blog. I ad love to visit it again and again. Thanks a bunch! Merely wanna say that this is very helpful , Thanks for taking your time to write this.
Yes, I am entirely agreed with this article, and I just want say that this article is very helpful and enlightening. I also have some precious piece of concerned info !!!!!!Thanks. It is my first visit to your blog, and I am very impressed with the articles that you serve. Give adequate knowledge for me. Thank you for sharing useful material. I will be back for the more great post. Nice post. I was checking constantly this blog and I’m impressed! Extremely useful info specially the last part I care for such information a lot. I was seeking this certain info for a long time. Thank you and good luck. 먹튀검증사이트
I have been exploring for a bit for any high quality articles or blog posts on this kind of area . Exploring in Yahoo I at last stumbled upon this site. Reading this information So i am happy to convey that I have a very good uncanny feeling I discovered exactly what I needed. I most certainly will make certain to do not forget this website and give it a look regularly. I truly wanted to write a brief message in order to appreciate you for all of the unique tricks you are writing at this site. My time intensive internet research has at the end been honored with good quality strategies to share with my best friends. I would declare that we readers actually are very lucky to live in a useful network with many brilliant individuals with beneficial suggestions. I feel very blessed to have seen your web pages and look forward to tons of more amazing moments reading here. Thanks once more for everything.
I’m really enjoying the design and layout of your site. won’t be a taboo subject but usually people are not enough to talk on such topics. To the next. Cheers 토토사이트
I’m really enjoying the design and layout of your site.
It’s fantastic. This is one of the top websites with a lot of useful information. This is an excellent piece, and I appreciate this website; keep up the fantastic work. Quite informative blog on how to bring an end to the issues. I like your creative blog and look forward to more insightful posts. I definitely enjoying every little bit of it. It is a great website and nice share. I want to thank you. Good job! You guys do a great blog, and have some great contents. Keep up the good work. Very good message. I stumbled across your blog and wanted to say that I really enjoyed reading your articles. Anyway, I will subscribe to your feed and hope you post again soon 온오프카지노주소
I found useful information on this topic as Now i’m focusing on a company project. Thank you posting relative information and its currently becoming easier to complete this project 토디즈
Good to become visiting your weblog again, it has been months for me. Nicely this article that i’ve been waited for so long. I will need this post to total my assignment in the college, and it has exact same topic together with your write-up. Thanks, good share. Pretty good post. I just stumbled upon your blog and wanted to say that I have really enjoyed reading your blog posts. Any way I’ll be subscribing to your feed and I hope you post again soon. 메이저놀이터
“Hi there! This post couldn’t be written any better! Looking at this post reminds me of my previous roommate! He always kept preaching about this. I’ll forward this article to him. Fairly certain he’s going to have a great read. Many thanks for sharing! Hey very cool web site!! Man .. Beautiful .. Amazing .. I’ll bookmark your web site and take the feeds also…I am happy to find a lot of useful info here in the post, we need develop more techniques in this regard, thanks for sharing. . . . . .
Wow! This can be one particular of the most useful blogs We’ve ever arrive across on this subject. Basically Wonderful. I’m also a specialist in this topic so I can understand your hard work. fantastic put up, very informative.” 먹튀
This is pretty well impressive thing I have found here. It looks cool and I agreed with the topics you just said. Thanks for the share.
Thank you for sharing this post
Students facing difficulty in writing assignment papers can take efficient assignment help from experts and sort out every paper query. Professionals know how to write top-notch solutions to complex assignment questions. Fetching good grades and meeting the deadline are guaranteed when you drop paper requirements to the experts.
First, shadows are the reflection of our existence in the space. Then, our shadows overlap, intertwine, communicate. In this project, shadows are turned into subjective via crypto representations of our mind states. Instead of being distorted by the source of light, the audience will be able to control their own shadows by wearing the headsets that collect EEG data from them and accordingly form fake shadows projected onto the ground beside them.
Very useful post, thanks for sharing.
I hope it will be helpful for almost all peoples that are searching for this type of topic. I Think this website is best for such topic. good work and quality of articles Thanks.
aomei partition assistant crack is an easy to use all-in-one Hard Disk Partition Software. AOMEI Partition Assistant 9.7.0 Crack offers various free partition management features for both all home users and commercial users. it guarantees the full features for creating, resizing, moving, copying, deleting, wiping, aligning, formating, merging, splitting partition, and more.
Thanks for Sharing Useful Information
Thanks for Sharing Useful Information
Very Interesting Article. Want to Know More
Quite an Interesting Article. Very Effective
Very Useful Trading Strategies.
Very Informative Blog. Everyone need this
Sounds Good and Effevtive
Lots of Thanks for Submission
MS Hydro fitments provide hydraulic pipe fittings manufactures that соme in а wide rаnge оf tyрes аnd аррliсаtiоns. We deliver high quаlity adaptors thrоugh highly skilled teсhniсаl cоnsultаnts, рrоviding the tyрe оf flexibility thаt ensures рrоjeсts run smооthly.
At THM we provide a rotary gear pump consisting of two meshing gear wheels in a suitable casing whose contra rotation entrains the fluid on one side and discharges it on the other. Buy best gear pumps at THM Haude.
Thank you for sharing such a nice and informative article with us. It was very interesting. Although this topic is usually interesting, your interesting writing makes it even more interesting. Thanks again for what you’ve done. New dresses deaign in pakistan.
I’ve read some good stuff here. Definitely worth bookmarking for revisiting. I surprise how much effort you put to create such a great informative website. SEO expert in karachi.
For years, PriceMyAC has been offering premium AC servicing and products throughout the Valley. We have completed hundreds of air conditioning system installations and repairs! Our experience and training allow us to promise that your air conditioner repair, service, or installation will be done correctly the first time. Call PriceMyAC, your local AC company in Phoenix, Tempe and nearby cities.
먹튀사이트
신규사이트
스포츠분석
Very useful post, thanks for sharing.
Very Interesting Article. Want to Know More
This is great
I want to always read your blogs. I love them Are you also searching for nursing dissertation writing services? we are the best solution for you. We are best known for delivering Nursing dissertation writing services to students without having to break the bank
congratulations. This is quite a good blog. Keep sharing. I love them Are you also searching for nursing writing help? we are the best solution for you.
Nice post thank you for sharing.
Thank you for sharing this post.
“Non-Fungible Tokens or NFT’s are digital assets that represent real-world objects like arts, music, in-game items, and videos. NFTs will revolutionize the way we look at them and open new revenue opportunities. Leverage the benefits of NFTs. Partner with our NFT development company to
expedite your development journey.”
I have never achieved more than 70% in my assignments. But with the support of great assignment help.com, I was able to achieve 93% in my assignments, and I would like to thank each and every professional in this assignment help Canada who has been a part of this experience. I would definitely love to work with the team again!
Students’ lives are burdened by the mountains of variable errands. These students are expected to accomplish their required assignments on the assigned date. But with variable other academic responsibilities, it becomes highly difficult to submit the following work on the mentioned deadline. Hiring a team of research paper writing help can assure steady and effective paper writing that can take a lot of your pressure.
We are equipped with multidisciplined <a href="Dissertation Help Experts“>dissertation help experts, who have the unique skills to write your dissertation. How do write a strong thesis statement, which is the first step in the entire research process? Our experts know, how to craft it. We are very particular about punctuation, grammar, and language & content while writing your dissertation. We are known as experts for our unique contributions in the field. We are not expensive!
Having been providing Management Assignment Help for longer than a decade now, our bespoke preparations are always plagiarism free and fully in sync with the latest criteria and rubrics of the faculty. We amplify and ensure your chances at the most excellent grades only.
I see your post and reed. This is a good for me this is a good post you give a grate information and helpful thanks for share this side. Check out list of top best VPS Hosting 2023 service providers that gives high-performance, 24/7 support & affordable VPS hosting price.
Visit W3Schoolsframeworks out there… Python Backtesting Libraries For Quant Trading Strategies […]
We furnish premium quality Electronics Engineering Writing Help to those in need at a very friendly price that suits a student’s pocket. We guarantee work free of plagiarism and grammatical errors always well before the stipulated deadline, thereby ensuring the best grade for you.
Thanks for sharing this, it’s really helpful…
UI UX Design Services
That’s why; take our efficient Assignment Help to write my paper online with the proper information. Our experts provide you the highly proven and well researched paper solutions that address every paper issues with the accurate data.
Good Job Admin! I just stumbled upon your weblog and wanted to say that I have really enjoyed browsing your blog posts. Check out my blog @
https://www.stiest.site/flo-and-kay-lyman-death
Thanks for sharing such a useful article here about python backtesting for quant trading strategies
Superb!!! Thanks for sharing this info.
Quant Trading Strategies Great write-up comparing the various python frameworks out there… Python Backtesting Libraries For Quant Trading Strategies […]
Thanks for sharing this useful information !
Thanks for sharing such a useful article here about python backtesting for quant trading strategies
Good points given are useful in many ways and useful to us in many ways.
Read More : nadi kuta meaning
Thanks for the blog
JBIT Institute dehradun , has remodeled their engineering programs on the philosophy of interdisciplinary learning. JBIT has opened up the doors for young minds who dare to dream.
Having been providing Criminology Assignment Help for longer than a decade now, our bespoke preparations are always plagiarism free and fully in sync with the latest criteria and rubrics of the faculty. We amplify and ensure your chances at the most excellent grades only.
Follow the instructions from our website to successfully download and install Brother Printer Drivers Download to enhance the current features of the printer.
Learn about the meaning of rocker finish tiles which will be useful to you and by knowing you can make many changes in the house.
Good information that is useful in many ways is useful for many businesses.
You also know how to strengthen the planets in your horoscope in life and what are the benefits of astrology remedies.
MS Hydro fitments are hydraulic pipe fittings manufactures that соme in а wide rаnge оf tyрes аnd аррliсаtiоns. We deliver high quаlity adaptors thrоugh highly skilled teсhniсаl cоnsultаnts, рrоviding the tyрe оf flexibility thаt ensures рrоjeсts run smооthly.
At THM we provide a rotary gear pumps consisting of two meshing gear wheels in a suitable casing whose contra rotation entrains the fluid on one side and discharges it on the other. Buy best gear pumps at THM Haude.
Amazing blog and valuable information. Thanks for putting so much effort in writing this article. UI UX Design Company
I’ve been looking for photos and articles on this topic over the past few days due to a school assignment, baccaratsite and I’m really happy to find a post with the material I was looking for! I bookmark and will come often! Thanks 😀
Assignments can provide tutor an opportunity to give every student specific feedback. Your feedback will help understudies in evaluating actual progress during the semester and can have a significant impact on their motivation to complete their education. Go to Assignment Help to take help of your subject assignment.
Good news about Python and we hope to add it to our website.
Visit our website and meet online doctor : https://meiracare.com/medication-prescription-refill-online
Square Quickbooks Integration Software sales invoices, multiple locations, taxes and discount.
Nice Post!! I really appreciated with you, Thank you for sharing your views with us.
Thank you for the information!!
hello friend video mai aapko bataya hun ki aap how to lose weight fast in 2 weeks exercise yar fir 2 weeks me vajan kaise kam kare for more information watch this video till end.
I’m very curious about how you write such a good article. Are you an expert on this subject? I think so. Thank you again for allowing me to read these posts, and have a nice day today. Thank you. 호치민 가라오케
Do you want to take Doctor note online to stay healthy or that too only, then you can visit our website for more information.
A thesis proposal is the outline of your upcoming ideas for your thesis paper. It is a document that defines your thesis topic issue and highlights the significance of your thesis topic. It elaborates why further research is essential for that specified field and proposes a significant solution for that issue. Thesis proposal is the prior step of transforming your thesis into a reality. The length of a thesis proposal varies from topic to topic. However, an average thesis proposal takes up to a minimum of 8 pages to 10 pages.
Warm day, my name is James Daniel! From the US. The QuickBooks error h202 is a difficult one to fix. However, with the appropriate solutions on your side, you can overcome these and other similar issues, allowing you to continue working on your QuickBooks financial transactions without interruption. Reach me, and I’ll go over all of the options for resolving this issue.
Can HP Printer Customer Service provide technical support?
Yes, HP Printer Customer Service provides technical support to its users. HP, a world-renowned brand in the tech world, offers useful information, features, and specifications about its range of products. The users can also get information about troubleshooting guides for their HP products.
Hi, I am Kanak rathorl. I am an educational consultant in an India-based company. If you are looking for all kinds of details about the future and education. After doing the Top mba colleges in pune you must have a look at our website. After 12th you will get very good information about the course. You will get all the details including exam preparation, syllabus, exam data.
Buy Car Engine Parts online, OEM Parts OEM Quality Parts in London Auto Parts Store. All Models include Audi, For our Engine products Call Now!
ibm spss statistics crack is a powerful tool used to analyze statistics and manage data and documents. It helps users quickly hold a significant business, social science, and many other relevant fields.
aomei partition assistant crack
Assignments for research papers must be completed by all college and university students. Writing research papers will be a requirement of your studies, whether you are pursuing a master’s or a bachelor’s degree. Research is required for courses and other activities in almost every topic. It does not change the reality that it could be difficult and stressful for the pupils, though. Instead of worrying over your academics, use our qualified research paper helper in the USA to find the shortest and easiest path to good scores. A number of academics with in-depth training and practical experience in the subject compose our staff of research paper writers. They can complete all of the demands that your teachers have outlined.
hitfilm express crack
ivt bluesoleil crack
We provide safe and secure payment options to the students. We are one of the most dependable Assignment Help service providers popularly known for offering top-notch quality assistance to students. In addition to this, students can make the payment in a hassle-free manner.
I really appreciate your efforts for this blog. This is so informative. Keep it up and keep sharing such posts.
Crafting an assignment a night before submitting is really a daunting task. Sometimes students fail to submit their assignments before deadlines due to multiple reasons. Sometimes they don’t have enough time to craft their assignment on their own because they are used to busy with their exam preparation. Therefore, they seek for someone to Do my assignment for me so that they can submit their assignment on time.
Thanks for sharing!
Great post
You posted very good content. thanx for this awesome content my site. We are Ph.D. professionals in Singapore, who are dedicated to providing you with help with your Assignment Help service at affordable rates and high quality.
Online assignment help in USA is a service that is provided to students who cannot manage to finish their assignment on time. assignment is usually given with deadlines attached to them. In countries like the USA, students often work and study simultaneously which can be stressful and quite difficult for them to manage at the same time.
Many people feel that they cannot get quality WordPress Development services as it is an open-source platform. It is a misconception as there is a huge community of developers involved with the development of this open-source platform. One such company providing high-quality WordPress Development services to its clients is Promanage IT Solutions.
Thank you for sharing your article with us, it means a lot to share information with anyone. I would like to say you that I am also here to share the information about online assignment help. We have a website, which name is Do Assignment Help. With Do Assignment Help, we offer online academic help for students. We live in Santa Clara, CA, USA, and provide online help worldwide.
Great post keep posting thank you!! outbooks
You gave fantastic honest ideas here. I performed a research on the issue and discovered that almost everyone is agree with your blog
You gave fantastic honest ideas here. I performed a research on the issue and discovered that almost everyone is agree with your blog
You gave fantastic honest ideas here. I performed a research on the issue and discovered that almost everyone is agree with your blogs
First of all, thank you for your post. baccaratsite Your posts are neatly organized with the information I want, so there are plenty of resources to reference. I bookmark this site and will find your posts frequently in the future. Thanks again ^^
You gave fantastic honest ideas here. Whatever the topic of your essay is, pick a best Argumentative Essay Topics that can produce good debate, so that you can create a solid base for all your arguments.
This surely helps me in my work. Lol, thanks for your comment! wink Glad you found it helpful. 메이저토토
Accounting & Bookkeeping Services for UK Accountants. Leading accounts outsourcing company with more than 20 Years of UK accounting experience. outsourcing for accountants
Of course, your article is good enough, baccarat online but I thought it would be much better to see professional photos and videos together. There are articles and photos on these topics on my homepage, so please visit and share your opinions.
Of course, your article is good enough, baccarat online but I thought it would be much better to see professional photos and videos together. There are articles and photos on these topics on my homepage, so please visit and share your opinions.
a
Great post keep posting thank you
Nice to meet you! I found your blog on msn. You’re so smart. I will add it to my favorites and read more of your posts whenever I have time. 토토사이트
“Don’t wait for the college professors to make your doubts clear, do it on your own with the help of the assignment help Canada service. We are eagerly waiting for your interesting requirements for the new assignments.”
Thanks for sharing such a wonderful information
Very good blog post, found it useful.
It often happens that students get anxious after getting so many assignments from their colleges and also submitting all those assignments on time is another reason behind getting anxious, so it is better to get some quality Management Assignment Help, for your assignment.
It often happens that students get anxious after getting so many assignments from their colleges and also submitting all those assignments on time is another reason behind getting anxious, so it is better to get some quality Management Assignment Help, for your assignment.
https://greatassignmenthelper.com/management-assignment-help/
Students can get top-notch quality International Trade Assignment Help from greatassignmenthelp.com at an inexpensive price. After using our services, students will earn an A+ academic grade at the most affordable price. Furthermore, students do not have to make a hole in their pocket since we give high-quality service at affordable pricing.
I’m writing on this topic these days, safetoto, but I have stopped writing because there is no reference material. Then I accidentally found your article. I can refer to a variety of materials, so I think the work I was preparing will work! Thank you for your efforts.
I have been looking for this kind of post for the last many days.Thanks for sharing it with us. This is a really great post.Can i ask if you find it all too perplexing to finish all your assignments on time?You can now relax.We specialize in reliable homework writing services and more course work services.Our team will always be by your side and assist you in the best way possible.Approach myhomeworkwriters.com the Most Trusted Assignment Help Website and take a step ahead towards your academic achievement!!
It is always advisable to complete the assignment well before the deadline and for that if you are self confident then its good but if not then go for aged care nursing assignment help
Your article has answered the question I was wondering about! I would like to write a thesis on this subject, but I would like you to give your opinion once 😀 majorsite
Accounts Payable (AP) is one of the most challenging tasks for businesses to perform in today’s competitive environment.
NFT Marketplace Development
TeraCopy Mac Crack Downlaod is a dynamic, personalized file copy program that allows you to pause and resume downloads, view destructive files bypassed at the end of the download, and find as much information as you need about each part of the file being downloaded. Copying multiple files can take a while on your computer, depending on how fast and good it is in terms of hardware.
By being embraced by January and the warm seeking, wisdom is much valued in the spring breeze. It gives and saves the spring wind, which is abundantly spring wind. Blood fades from youth, and youthful skin is the spring breeze. If not called to them, I am glad, giving, and with myself. There are all kinds of things, and soon to come. Yes, live happily and at the end of the fruit how much your heart will be on ice. Even if you search for it, the magnificent blood of love holds a large hug, what do you see here. It is a bar, from the days of January and the Golden Age.비아그라구매 For the sake of ideals, it is a bar, it will. Decaying beauty hardens, and bright sandy birds hold onto it.
Good!!!
Solana NFT Marketplace Development
Great post, visit our site today.
☆죽지 않는 남편의 力☆
【조루男 #정품비아그라 먹고 성관계 ☆바람난 아내도 깜놀 】
☎ 카톡: Kman888
♥온라인 No.1정품판매 업체♥
♥처방전없이 구매가능♥
사이트 바로가기 >>https://kviaman.com
Don’t hesitate in looking out for support from a reliable Tort Law Assignment Help to ensure good academic grades because these grades often define your career graph. In today’s world of hugely demanding academic life, there’s nothing wrong in seeking help which often proves to be a very wise call if taken timely.
It’s the same topic , but I was quite surprised to see the opinions I didn’t think of. My blog also has articles on these topics, so I look forward to your visit. safetoto
Philosophy is an important part of a degree course. Many students need help in philosophy dissertations to increase their scores. Our experts offer online philosophy dissertation help service for scholars. With the help of this service, you can get high-quality and error-free dissertations. If your dissertation is unique and informative you can get your teacher’s favor.
QuickBooks is the biggest accounting software in the world. One of the most common error series in QuickBooks is the “H” series. we’ll tell you How to fix Error Code H505. If you’ll read this blog, then it means you’ll definitely solve this error.Visit our website
Hi companions ! If you have any desire to get the best assistance, then you can agree with it from our position, which our side gives the best help. Gives different sorts of administration.
Hi companions ! If you have any desire to Raipur Escorts get the best assistance, then you can agree with it from our position, which our side gives the best help. Gives different sorts of administration.
Thanks for sharing informative information.
https://herons.com.au/
I like these topics very much. I would like to see such topics daily, this post is very good indeed. There are people like you in the world who put forth their views. Thank you so much for posting such a great post.
I follow your posts. if you need help with papers, I will be very glad to help you. My service Feel free to contact me at any time.
After reading several of your blog postings, I came to the conclusion that this website gave guidance. reposition the setup
I love seeing blogs that understand the value.Amritsar Escorts I’m glad to have found this post as its such an interesting one!
Know about our blog about NFTs Web3 gaming which will be useful for you and also work in money invest.
Thanks for sharing informative information !
I love seeing blogs that understand the value of http://www.escortsludhiana.in/amritsar-escorts.html I’m glad to have found this post as it’s such an interesting one!
I love seeing blogs that understand the value of http://www.escortsludhiana.in/amritsar-escorts.html I’m glad to have found this post as it’s such an interesting one!
I love seeing blogs that understand the value of http://www.escortsludhiana.in/
Without a possibility of a doubt, a pupil has a plethora of difficulties to deal every day. Maintaining concentration and making the extra-effort that each online program requires can become challenging and stressful. Because of a lack of concentration and drive, students frequently quit their classes, receive below-average marks, or even flunk the test. This is the reason “ write my paper”, is providing its prized pupils with the best services possible. With everything taken care of for you, you will not have to worry about your writing paper, projects, assignments, exams, essays, difficult quizzes, or even the written test. Simply get in touch with them and compose a piece on your own achievements to ensure an A or B grade.
Pretty! This was a truly great article.
Escorts in Bangalore Gratitude for providing this data.
Thank you for this wonderful post! It has long been extremely helpful. escorts in mahipalpur I wish that you will carry on posting your knowledge with us thanks for sharing this post.
Thank you for this wonderful post! It has long been extremely helpful. I wish that you will carry on posting your knowledge with us thanks for sharing this post.
Hi,
This is really a nice blog by you. I really appreciate your efforts for this blog. Keep it up and keep posting such blogs.
Education plays an important role in every person’s life. Without proper education you can’t expect a bright future. This is the reason why every parent asks their children to study properly. But not every child has much interest in study. Sometimes we should develop the interest of our child towards studies so that they will themself start studying. In the world of digitalization, Online tuition is the best way to develop interest in students’ studies. You can take online tuition for class 8, 9, 10 or for any class for your child and see your child growing.
Your pearl is staggering and I close by you and skip for a surprisingly long time enlightening posts. Escorts Service in Mumbai Appreciative to you for developing shocking information with us
Great information is shared in this article. I simply suggest you to publish more articles like these. Its really an interesting blog.
Great information is shared in this article. I simply suggest you to publish more articles like these. Its really an interesting blog. Now its time to avail toughened glass shopfront for more information.
This article has been of great help to the topic I was looking for for an assignment. Thank you for your posts and I’d love to see them often. 토토사이트
Look for sex stories wonderful sexual tales. I found this wonderful article of yours, thank you.
Delivering help with nursing assignment at UK becomes more crucial because students and professors look forward for the dedication in the subject matter. The assignments given to the nursing students require them to discover new facts and knowledge that will help them improve their skills as nurses. When it comes to a student’s grades, assignments have a significant role. In the form of projects and assignments, most nursing schools assign a wide range of themes and concepts. Our experts at greatassignmenthelp.com can add lot of value in your assignment at best price to save time which can be spared for studies.
Thanks for sharing this information. I really like your blog post very much. You have really shared an informative and interesting blog post with people
“Are you sad because you got poor marks last year because you couldn’t deliver your assignments on time? Don’t worry anymore! Because Assignment Help Windsor agencies have come up with ultimate support for you.”
A few days ago, my sister purchased this Falling for Christmas Lindsay Lohan Apres Ski Sweater from jacket-hub for my mother. This Christmas, she made the decision to give this sweater. She purchased it in a matter of days for the lowest cost.
First of all, thank you for your post. slotsite Your posts are neatly organized with the information I want, so there are plenty of resources to reference. I bookmark this site and will find your posts frequently in the future. Thanks again ^^
I am so glad for found this blog in my search result page. This is very wonderful blog. Escorts Service in Chennai
Thanks for sharing.
People think that they do not require Online Assignment Helpfor their assignments but they don’t understand the value of this because any online assignment help site can help you in the perfect way because they have the expertise in their domain and they know that how to handle an assignment or what is the required way to do an assignment so that you can get quality grades or the grades that you want to achieve with the help of this.
Thanks for sharing!
Thanks for contributing a piece of valuable details. If you have trouble logging in with Quickbooks, you can fix it with the help of the Quickbooks File Doctor tool also contact Quickbooks payroll support.
Thanks for contributing a piece of valuable details. If you have trouble logging in with Quickbooks, you can fix it with the help of the Quickbooks File Doctor tool also contact Quickbooks payroll support.
I’ve been looking for photos and articles on this topic over the past few days due to a school assignment, baccarat online and I’m really happy to find a post with the material I was looking for! I bookmark and will come often! Thanks 😀
I’ve been looking for photos and articles on this topic over the past few days due to a school assignment, baccarat online and I’m really happy to find a post with the material I was looking for! I bookmark and will come often! Thanks 😀
125
Thank you for sharing such a piece of beautiful information
I came to this site with the introduction of a friend around me and I was very impressed when I found your writing. I’ll come back often after bookmarking! bitcoincasino
I am so lucky to finally find this article. I am a person who deals with kind of this too.토토사이트추천 This article has huge information for me, and well prepared for people who need about this. I am sure this must be comepletly useful for many people in the world.
This product has many features. and you also try links to know about all features of this product
Hello! This is my first visit to your blog! We are a group of volunteers and new startups in the same area. The blog provided useful information to work on. You did a great job!
Hello! This is my first visit to your blog! We are a group of volunteers and new startups in the same area. The blog provided useful information to work on. You did a great job!
While reading the article, I wondered how to write such an article. Looking at your good writing, I want to inform others and contact others.https://totomeoktwiblog.com/
I think you are amazing for maintaining a site like this. It’s not easy. You are also a great person.먹튀검증
I read the article for a while and checked the good parts. Not only this checked part, but many articles have good information.메이저사이트
I’m happy to have a break-like time on your site with a lot of new information and stories. Thank you. 안전놀이터
Awesome Blog!!! Thanks to Admin for sharing the above list. I visited many pages of your Blog. Really your Blog is Awesome. Keep Sharing such good Stories. Thanks. Visit Here: Opal
Really your information is Awesome. Keep Sharing such good Stories. Thanks. Visit Here: Opal
I read your post and I was impressed by your post a lot. You keep posting like this. Thank you for Sharing.
Hello There. I found your blog using google. This is a very well written article. I’ll be sure to bookmark it and come back to read more of your useful information. Thanks for the post.
Hello I found your article using google. This is a very well written article. I’ll be sure to bookmark it and come back to read more of your useful information. Thanks
I read your post and I was impressed by your post a lot. You keep posting like this. Thank you for Sharing. If you want write for us please visit here: write for us technology
They have been carefully selected just for you so you can enjoy every moment with them. If you have never tried an escort before, we provide 100% satisfaction guarantee because it is impossible to stay unsatisfied after such a
fabulous experience.
Your article is on an exceptionally essential level reasonable and unbelievably gigantic, the substance is unfathomably reasonable and incredible.
It’s too bad to check your article late. I wonder what it would be if we met a little faster. I want to exchange a little more, but please visit my site totosite and leave a message!!
Great article and a nice way to promote online. I’m satisfied with the information that you provided.
In today’s article, we will be talking about the QuickBooks Error Code 6175, 0. This article is written with an aim to provide an in-depth knowledge regarding this error.
I was looking for an article then I found an article, I enjoyed a lot after reading that article. I want you to read this article once. Gurgaon Women Looking For Men
I was looking for an article then I found an article, I enjoyed a lot after reading that article. I want you to read this article once.
I appreciate how you display your stuff. If anyone needs Delhi Escort assistance presenting their schoolwork in an appealing way, let us know.
QuickBooks is a financial software package that helps small and medium-sized businesses manage their finances, including payroll, billing, and tax preparation. QuickBooks offers a number of different products, including QuickBooks Online, QuickBooks Enterprise, QuickBooks Payroll, QuickBooks Pro, and QuickBooks Premier.
Thanks for a great article. Where else could I get this kind of information in such an ideal method of writing? I’m a search of such info for presentation
I found your article using google. This is a very well-written article. I’ll be sure to bookmark it and come back to read more of your useful information.
Thanks for sharing, really i need this
Thanks for sharing, really i need thiss
Online exam preparation has become very common due to the quick development of technology. Students can obtain individualized aid in exam preparation by using online exam help resources. Students can better learn the subjects and develop confidence in their abilities with the aid of these resources, which also provide in-depth information and practice exams.
Awesome Information!!! Thanks to Admin for sharing this. I visited many pages of your website. Really your Blog is Awesome. Keep Sharing such good Stories. Thanks.
These individuals enjoy their work and often create a safe space for their clients who may not feel comfortable or secure with other individuals.
The best way to find the perfect escort near you is to do a search online. You can use websites like Escort-Ads.com or other escort websites to search for local escorts in your area
Very helpful advice in this particular post! It’s the little changes that make the largest changes. Thanks for sharing! visit here: Turquoise jewelry
Indulge yourself in the luxury of the tropics by styling soothing Larimar Jewelry. The stunning light blue gemstone looks precisely like the waves of the sea. Tempting Larimar is a hidden jewel that seizes the beauty of the Caribbean Islands. Style alluring Larimar pendants and rings for calming vibes. Explore the enticing range of Larimar gemstone ornament at the site of Rananjay Exports, as they are the top producer and supplier of wholesale gemstone jewelry.
I have enjoyed reading your blog . As a fellow writer and Kindle publishing enthusiast, I would like to first thank you for the sheer volume of useful resources you have compiled for authors in your blog and across the web.I’m Alos work on blog i hope you like my Amethyst Jewelry blogs.
fantastic Blog !!! Thanks to Admin for sharing the above list. I visited many pages of your Blog. Really your Blog is very Awesome. Keep Sharing such good Stories. Thanks.
Being a long-time reader at this point, I feel that I have a pretty good grasp on the type of stuff your readers might enjoy and find helpful. However, I was just hoping to touch base with you to find out what you think would be best..I’m Alos work on blog i hope you like my Amethyst Jewelry blogs.
amazing Blog !!! Thanks to Admin for sharing the above list. I visited many pages of your Blog. Really your Blog is very Awesome. Keep Sharing such good Stories. Thanks you
Thanks for sharing excellent information. Your website is so cool. I am impressed by the details that you have on this website. It reveals how nicely you understand this subject. Keep sharing posts like this.
Fantastic Blog !!! Thanks to Admin for sharing the above list. I visited many pages of your Blog. Really your Blog is Awesome. Keep Sharing such good Stories. Thanks.
Fantastic Blog !!! Thanks to Admin for sharing the above list. I visited many pages of your Blog. Thanks.
Awesome post! You are sharing amazing information through your blog. I am a big fan of your excellent writing skills. Keep Sharing such good Stories.
One of the things I love about Moonstone Jewelry is its versatility. It pairs beautifully with both casual and formal wear, and its soft, neutral color complements any skin tone. Plus, there are so many different designs and styles to choose from, so you can find a piece that suits your personal taste and style.
This is really a great post have you written.I
This is really a great post have you written.I really enjoyed
Amazing post!! You are fabulous
I read your post and I was impressed by your post a lot. You keep posting like this. Thank you for Sharing.
fastastic Blog !!! Thanks to Admin for sharing the above list. I visited many pages of your Blog. Really your Blog is Awesome. Keep Sharing such good Stories. Thanks.
fastastic Blog !!! Thanks to Admin for sharing the above list.
fastastic Blog please check my link
Very nice Blog please check my link
Thanks for the nice blog. It was very useful for me. I’m happy I found this blog. Thank you for sharing with us, I too always learn something new from your post.
I am very happy to discover your post as it will become on top in my collection of favorite blogs to visit.
You will feel happy to realize that our Jaipur Escort will give you the most gorgeous and rich women who are experts in their work. They are knowledgeable and respectful young ladies who give certified escort administrations to their clients. Accompanies in Jaipur are high-profile models who are popular for their quality escort administrations.
Here you can find a wide range of female models like Russian Call Young ladies, Models, Big name Escorts, Educators, Housewives, and School accompanies in Jaipur accessible consistently at reasonable rates.
Nice Blog !!! Thanks to Admin for sharing the above list. I visited many pages of your Blog. Really your Blog is Awesome. Keep Sharing such good Stories. Thanks.
Thanks to Admin for sharing the above list. I visited many pages of your Blog. Really your Blog is Awesome. Keep Sharing such good Stories. Thanks.
Enjoyed reading the article above , really explains everything in detail, the article is very interesting and fashionable. Thank you and Wish to see much more like this.
Thank you so much for sharing such useful information over here with us. This is really a great blog have you written. I really enjoyed reading your article. I will be looking forward to reading your next post.
This Amethyst Stalactite-2SP (ASL-1-7) is a beautiful piece of jewelry that will be the perfect partner for a delicate neckline. Including this pendant in the jewelry collection will be one of the best decisions anyone can make. Wearing this pendant on special occasions will add the vibe of the wearer’s personality to the overall appearance. It becomes the head turner amongst the masses when flaunted with style and elegance.
I read your blog and learned a lot from it, I was looking for such a blog, and many thanks for sharing this information.
Thank you so much for sharing such useful information over here with us. This is really a great blog have you written. I really enjoyed reading your article. I will be looking forward to reading your next post.
https://www.rananjayexports.com/gemstones/moldavite
You have written a very good article, I got a lot of pleasure after reading this article of yours, I hope that you will submit your second article soon. Thank You
I read your blog and learned a lot from it, I was looking for such a blog, and many thanks for sharing this information.
great Blog !!! Thanks to Admin for sharing the above list. I visited many pages of your Blog. Really your Blog is Awesome. Keep Sharing such good Stories. Thanks.
You have written a very good article, I got a lot of pleasure after reading this article of yours, I hope that you will submit your second article soon. Thank You
Awesome Blog!!! Thanks to Admin for sharing the above list. I visited many pages of your Blog. Really your Blog is Awesome. Keep Sharing such good Stories.
Visit Abu Road Escorts and be seduced by its exotic escorts
Once you begin to explore its main sites of interest, you can’t fail to realise that you’re looking at somewhere very special.
Book Call Girls on https://www.depika-kaur.in/abu-road-escorts
Nice Blog!!! Thanks to Admin for sharing the above list. I visited many pages of your Blog. Really your Blog is Awesome. Keep Sharing such good Stories. Thanks.
Larimar belongs to the Caribbean region of the Dominican Republic. The larimar gemstone ring is popular due to its stunning bluish existence on its surface and contains powers of the sky and ocean elements. Larimar was first found by a stone artisan Miguel Mendez near the seashore, and he put the crystal’s name on his daughter’s name Larissa.
Very nice information related to this Blog. This Information is very good and helpful, Thank you to provide us.
Thank you for sharing your valuable article with us, it means a lot. I like your content too much because it provides us with information as well as knowledge. Like you, I am also here to tell everyone, especially students about my essay-writing website which is Write My Essay. With this website, we provide academic content to the students like essays, dissertations, coursework, and other types of content. So if you are doing your essay and feel difficult then you can contact our Write My Essay experts for help. They will help you best according to your issues. End I would like to tell you that I and my team live in Santa Clara, USA, and provide essay writing help online. For more information, you may contact us through our website.
Thanks to share this post about Python Backtesting libraries. i like your post.
Thank you………
Thanks to share this post .
Thank you………
925 Silver Shine have the amazing team for silver jewelry, Semi-Precious Gemstone and Silver Findings Manufacturers. We create the designs as per the trends and sales to wholesale buyers. If you are looking for the wholesale silver jewelry creative designs, then you are on the right place of the web. The best Silver Jewelry Wholesaler & supplier of 925 silver jewellery online is here. You will get the most amazing experience of buying silver Jewelry Such as Gemstone Silver Rings, Gemstone Silver Earrings, Gemstone silver Pendant Etc.
This Amethyst Stalactite-2SP (ASL-1-7) is a beautiful piece of jewelry that will be the perfect partner for a delicate neckline. Including this pendant in the jewelry collection will be one of the best decisions anyone can make. Wearing this pendant on special occasions will add the vibe of the wearer’s personality to the overall appearance. It becomes the head turner amongst the masses when flaunted with style and elegance.
This Amethyst Stalactite-2SP (ASL-1-7) is a beautiful piece of jewelry that will be the perfect partner for a delicate neckline. Including this pendant in the jewelry collection will be one of the best decisions anyone can make. Wearing this pendant on special occasions will add the vibe of the wearer’s personality to the overall appearance. It becomes the head turner amongst the masses when flaunted with style and elegance.
Thanks For Sharing About Python.
I have read so many posts on the topic of the blogger lover but this paragraph is genuinely a please, keep it up.
Loved the blog.
I’ve been reading about your blog and topic for the past two days, and I’m still bringing it! It was very effective to wonder about your words in each line. https://totoxetak.com/
if you are facing QuickBooks Migration Failed Unexpectedly’ is a technical problem in which the QB users fail to migrate the QB Desktop to another computer. But you don’t. I will tell you how you can do all this very easily. can do from, You can contact me at once by dropping me an email or via live chat. I am accessible at all times. I am here to assist you and 24/7 available for your support.
Hi everyone, I am Mac Davis as your assistant if you are searching for How to Write QuickBooks Direct Deposit Form so don’t worry I am telling you how to access QB Direct Deposit Form within a few minutes. So, I’m here to answer any QuickBooks questions you may have. I’ve been working with QuickBooks for a long time. in case you have any questions, I’m here to help.
Dumpor is still the top app of its sort despite this. We never keep any of your information on Dumpor’s servers because they don’t have a logging policy.
Tere Ishq Mein Ghayal Is Indain Tv Drama By Colors tv Tere Ishq Mein Ghayal is Hindi Serial Tv
Tere Ishq Mein Ghayal
Wonderful blog! A variety of cutting-edge startups, ideas, and other topics are covered by Tech City Studio. you’re welcome to write for us tech.
Inrecidble blog! A variety of cutting-edge startups, ideas, and other topics are covered by Tech City Studio. you’re welcome to write for us tech.
Awesome blog. you’re welcome to write for us tech.
Such a great article! thank you for sharing the valuable knowledge.
Your blog has become one of my go-to resources for information and inspiration. Thank you for your hard work and dedication to creating such valuable content
For Blockchain Development Services
visit:https://www.metappfactory.com/blockchain-development-service/
Assignment helpers and assignment help providers in Canada aim to provide high-quality assignments to the students belonging to different universities and help them achieve high grades. assignment
Help Canada is known to be one of the most educated countries with about 60% of the population having received a post-secondary degree.
I’m writing on this topic these days, baccaratcommunity, but I have stopped writing because there is no reference material. Then I accidentally found your article. I can refer to a variety of materials, so I think the work I was preparing will work! Thank you for your efforts.
Really very nice blog. Checkt this also- Best dog groomer near me
Are you in Ireland and struggling with your online exams? Look no further! We offer expert assistance for “do my online exam Ireland” requests. Our team of skilled professionals is here to help you excel in your academic pursuits. From taking your online exam to ensuring that you receive top grades, we have got you covered. Trust us to provide reliable and affordable services that meet your needs.
I’m writing on this topic these days, slotsite, but I have stopped writing because there is no reference material. Then I accidentally found your article. I can refer to a variety of materials, so I think the work I was preparing will work! Thank you for your efforts.
Why couldn’t I have the same or similar opinions as you? T^T I hope you also visit my blog and give us a good opinion. casino online
I was looking for another article by chance and found your article casino online I am writing on this topic, so I think it will help a lot. I leave my blog address below. Please visit once.
When I read an article on this topic, bitcoincasino the first thought was profound and difficult, and I wondered if others could understand.. My site has a discussion board for articles and photos similar to this topic. Could you please visit me when you have time to discuss this topic?
When I read an article on this topic, majorsite the first thought was profound and difficult, and I wondered if others could understand.. My site has a discussion board for articles and photos similar to this topic. Could you please visit me when you have time to discuss this topic?
I have been looking for articles on these topics for a long time. totosite I don’t know how grateful you are for posting on this topic. Thank you for the numerous articles on this site, I will subscribe to those links in my bookmarks and visit them often. Have a nice day
South Delhi Escorts is one of the best options for escorts in South Delhi. There are many reasons for you to hire a VIP South Delhi Escorts, but we will look at the top three below.
If you are getting errors while connecting to QB software to database, QuickBooks Connection Diagnostic Tool can help you in this.
your are a great author It’s contains many informative content Attorney Bankruptcies
keep write more article like this
useful article
1st birthday wishes for baby boy from parents you can more images like this in my blog,
useful article
prenuptial agreement you can more images like this in my blog,
Look up How do I Talk to a Live Person at Air France. This is typically available on the airline’s website, Making it easier for the representative to find your account and help you more quickly.
You always have the option to browse around till you find a better Deal If You Want to know the offers they present and you find out how to talk to someone on delta airlines so this is the Right Place Where You Got Excellent deals.
I just found this blog and I really hope it continues. Keep up the good work, nice people are hard to find. I have added to my favourites. Thanks. Please see my blog.Dhytexasbuilders.comBathroom Remodeling Contractors Houston
A Short Wolf Cut With Bangs is a trendy and edgy hairstyle that is popular with women. The wolf cut is a layered haircut that adds volume and texture to short hair, with the top layers being shorter than the bottom layers. Adding bangs to the cut can add even more dimension to the style, and there are a few ways to incorporate bangs into a wolf cut. For example, you can opt for curtain bangs that frame the face, or choppy and textured bangs for a more dramatic look.
I thoroughly read this article regarding the differences between the most recent and Latest Technology News
accidente de moto maryland
Thanks for sharing the blog’s information and it’s importance. The content is very useful and informative.
Thank you for sharing this informative article on Binance clone script development. It’s great to see that there are reliable and trustworthy companies like Opris that specialize in providing Binance clone script development services. With their expertise and experience, they can help entrepreneurs and businesses to quickly launch their own cryptocurrency exchange platform with all the necessary features and functionalities. I would like to recommend adding Opris to the list of Binance clone script developers. They have a readymade Binance clone script that can help save time and costs for those looking to start their own exchange platform. Keep up the good work and thanks for sharing this valuable information
Binance Clone Script
I just couldn’t evaporate from your site before suggesting that I really secured a couple of excellent encounters the standard data an individual obliges your guests? Will be again perpetually to look at new posts.
I must say. Rarely do I encounter a blog that’ѕ both equallу eԁucatіvе and interesting, аnd withоut a dоubt, уou hаve hit the nail on the head.
Simply continue visiting this website to stay informed of the Top Trending News updates posted here if you want to improve your experience.
Hello ! I am the one who writes posts on these topics bitcoincasino I would like to write an article based on your article. When can I ask for a review?
Thanks you for sharing this amazing content it was really helpful and informative. I will surely share it with my friends. Keep it up.
I found your blog using google. This is a very well-written article. I’ll be sure to bookmark it and come back to read more of your useful information. Thanks for the post
I Hope You Will Share Such Type Of Impressive Content Again With Us So That We Can Utilize It And Get More Advantage.
Casino Highlights – VIP Blackjack, 바둑이게임 and Regular Blackjack
If you booked your ticket from Alaska Airlines and now you want to cancel your ticket due to some reason but don’t know the Alaska Airlines cancellation policy, then no need to worry I have a list of cancellation policy and you can check this cancellation policy and save your money upto 50%.
thank you
If you are seeking UAE assignment help, you’re in the right place. Our team of experienced professionals is dedicated to providing top-notch assistance to students in the UAE. Whether you need help with research papers, essays, presentations, or any other assignment, we offer comprehensive support tailored to your specific requirements. With our expertise and knowledge, we ensure high-quality work that meets academic standards. Don’t let assignment stress overwhelm you—reach out to us for reliable UAE assignment help and excel in your academic endeavors.
Assignment Help services have been instrumental in my academic success. They have provided me with the necessary support, guidance, and resources to overcome challenges, improve my grades, and enhance my overall learning experience. I highly recommend assignment help services to any student who finds themselves struggling with the demands of academic life. It is a decision that can positively impact your academic journey and pave the way for future success.
Emotive language refers to the use of words, phrases, or expressions that are specifically chosen to elicit an emotional response from the listener or reader. It is a type of language that aims to evoke strong feelings, opinions, or reactions by appealing to the emotions and personal beliefs of the audience.
Emotive language is commonly used in various forms of communication, such as persuasive writing, speeches, advertising, or political discourse, to influence or sway people’s opinions and attitudes. By employing words that evoke specific emotions, the speaker or writer seeks to create a powerful connection with the audience and shape their perception of a particular topic or issue.
Abortion is a highly debated and controversial topic, with many people holding strong and opposing views. However, here are some reasons why abortion should be legal.
Women’s right to choose: A woman should have the right to make decisions about her own body and health, including whether or not to have an abortion. Restricting access to safe and legal abortion services violates women’s rights and autonomy.
https://myassignmenthelp.com/blog/why-abortion-should-be-legal-essay/
How To Write A Counter Argument ?
Arguments and counterarguments are The vehicles for delivering an oratorial grand slam. Few weapons in a skilful orator or writer are as effective as clear, logical, and evidence-backed arguments and counter arguments.
Whereas arguments communicate standpoints & the reasons why one supports them, counterarguments refute and reinforce the preceding arguments of a class. Developing the perfect counterargument that trumps and stuns the opposition is a skill. This article will dig deep into all it takes to craft clear & decisive counterarguments.
it was great post thanks for this : BookMyEssay offers professional Dissertation Methodology Writing Help, ensuring thorough research, data collection, and analysis. Our expert writers provide valuable insights and guidance, ensuring high-quality and well-structured dissertations. Trust us for your academic success.
Great post! Thanks for sharing
pgslot vip PGSLOT AUTO เล่นง่ายขึ้น แตกง่ายมาก ทำเงินได้ในทุกวัน
Thanks for sharing beautiful content. I got information from your blog. keep sharing. I need more information about this blog. I am new in the blog writing. its very helpful for me
As a leading Metaverse App Development Company, we can turn your concepts into reality, offering an interactive experience for your clients with a range of features and functionalities.
Thanks for the great tips. Now my work is very easy for the reply to the reviews. I implement it right now.
Thanks for sharing superb informations. Your web site is very cool. I’m impressed by the details that you have on this blog, for leather jacket in discounted prices you can visit our website and get the leather jacket in discounted prices
deunoposte ojogodobicho” é um blog que publica rapidamente os resultados do Jogo do Bicho após cada apuração
Long after the show is over you will still be thinking about this incredible evening of Party Entertainment Ideas
Are you frustrated with Java homework? Do you need instant Java help? Get instant Java programming help from Java experts at a 30% Off discount. Order Now!
Java Programming Help
Thank you for the auspicious writeup. It in fact was a amusement account it. https://mtline9.com
This is a great opportunity to visit such a site and I’m glad to know about it. Thank you for giving us the opportunity to take advantage of this opportunity Furniture in Karachi
Global Assignment Help provides high-quality Assignment Help in the USA. They have experienced writers, offer affordable pricing, and ensure high-quality work. They also provide round-the-clock customer support and timely delivery of plagiarism-free work.
Do you find yourself struggling with your online marketing assignment? Fret not, because our dedicated team is here to lend you a helping hand. At our online platform, we offer impeccable online marketing assignment help services tailored specifically to meet your requirements. Whether you are facing difficulties with market analysis, digital strategy, or any other aspect of online marketing, our experts will guide you through every step to ensure your success. Let us lighten your workload and pave your way to academic excellence!
Top Follow Apk is a popular social media application that allows users to gain followers, likes, and comments on their Instagram accounts. With the
Top Follow MOD APK download, users can enjoy unlimited coins and access to a wide range of features.
If You Are Confused about How Grab American Airlines Last Minute Deals You Are In Right Place Aairtickets Guide To Take Discounted Tickets For You vacation And Business Trips.So Why You are Waiting Just Click On the Deal And Take Affordable Deals on American Airlines.
I found it worth reading. I just want to ask you to write more Best Jewellery Design
It’s actually a great and helpful piece of information. I’m satisfied that you shared this useful info with us. Please stay us informed like this. Thank you for sharing.
Students who struggle to pay attention in class or who are reluctant to ask their teachers pertinent questions could benefit from expert assignment help UAE to ensure they receive high grades on their assignments. A pupil won’t be able to accomplish their job successfully if they don’t have access to transparency on their subject matter. A student can submit their project on time by using Assignment Help in UAE, and by afterwards reading the simple language-written materials, they will have all the clarification they require to comprehend the challenging subject matter.
Students who struggle to pay attention in class or who are reluctant to ask their teachers pertinent questions could benefit from expert assignment help UAE to ensure they receive high grades on their assignments. A pupil won’t be able to accomplish their job successfully if they don’t have access to transparency on their subject matter. A student can submit their project on time by using Assignment Help in UAE, and by afterwards reading the simple language-written materials, they will have all the clarification they require to comprehend the challenging subject matter.
Best Pakistani and Indian Dresses in Chicago
Keep it up. I really like your content, I have forwared it to my facebook feeds and many followers like it.
Thanks for sharing beautiful content. I got information from your blog. keep sharing. I need more information about this blog. I am new in the blog writing. its very helpful for me
It truly useful & it helped me out much. I hope to give something back
and help others like you Digital marketing agency
Best Pakistani and Indian Dresses in Chicago It’s really a cool and useful piece of information. I’m glad that you shared this useful information with us. Please keep us informed like this. Thanks for sharing.
I must say. Rarely do I encounter a blog that’ѕ both equallу eԁucatіvе and interesting, аnd withоut a dоubt, уou hаve hit the nail on the head.currently we are working on gemstone such as peridot ring if you want any information contact us.
I must say. Rarely do I encounter a blog that’ѕ both equallу eԁucatіvе and interesting, аnd withоut a dоubt, уou hаve hit the nail on the head.currently we are working on gemstone such as citrine ring if you want any information contact us.
I must say. Rarely do I encounter a blog that’ѕ both equallу eԁucatіvе and interesting, аnd withоut a dоubt, уou hаve hit the nail on the head.currently we are working on gemstone such as peridot jewelry if you want any information contact us.
I must say. Rarely do I encounter a blog that’ѕ both equallу eԁucatіvе and interesting, аnd withоut a dоubt, уou hаve hit the nail on the head.currently we are working on gemstone such as peridot rings if you want any information contact us.
Get latest voucher codes, deals and offers only at:
Voucher Codes
Obtenga los últimos códigos de cupones, promociones y ofertas solo en:
Cupon Codigo
It truly useful & it helped me out much. I hope to give something back
and help others like you best designer jewelry
If Want To Know How Spirit Low Fare Calendar Works For Travller Who Want Affordable Tickets For Vacation So You Are In Right Place Wher You Get The Latest Deals On Spirit Airlines
It is essential to backtest quant trading strategies before trading them with real money. Here, we review frequently used Python backtesting libraries. We examine them in terms of flexibility (can be used for backtesting, paper-trading as well as live-trading), ease of use (good documentation, good structure) and scalability (speed, simplicity, and compatibility with other libraries).
Assignment Help Online USA services prioritize the confidentiality of student information and maintain a secure platform for communication and data exchange. Students can feel comfortable sharing their academic concerns and requirements, knowing that their privacy is protected. Additionally, these services offer round-the-clock customer support, allowing students to seek assistance and clarification at any time, further enhancing their overall experience.
This is a great opportunity to visit such a site and I’m glad to know about it. Thank you for giving us the opportunity to take advantage of this opportunity Furniture in Karachi
Python backtesting libraries offer a range of features and functionalities that make it easier for traders to test and validate their quantitative models. These libraries provide tools for data manipulation, strategy development and evaluation, risk management, and portfolio optimization.
If you are looking for online class help then visit our websites. Onlineclasshelp.io can be a valuable resource for students seeking additional support and guidance in their academic pursuits. With the advancement of technology, online platforms have made it easier than ever for students to access educational resources and assistance from the comfort of their own homes.
I’m a blogger that enjoys reading interesting blogs like yours.
This is a great opportunity to visit such a site and I’m glad to know about it. Thank you for giving us the opportunity to take advantage of this opportunity Mobile accessories price in Pakistan
This is a great opportunity to visit such a site and I’m glad to know about it. Thank you for giving us the opportunity to take advantage of this opportunity Smart watch price in Pakistan
First time visiting this place. Judging by the numerous comments on your article, I guess I wasn’t the only one having all the fun here! Please visit my blog too 😉먹튀오버
Get inspired and discover how companies are implementing the multimodal GPT-4 model to power new AI use cases. Visit GPT AppX to explore the possibilities.
This is a great opportunity to visit such a site and I’m glad to know about it. Thank you for giving us the opportunity to take advantage of this opportunity Power Bank price in Pakistan
Hello, friends. This is Emma. I frequently travel. I visited numerous locations. You can check here to learn more about recent travel news. what terminal is jetblue at logan airport
Welcome to the world of 0 nicotine disposable vapes, where you can indulge in the rich flavors and satisfying sensation of vaping without any nicotine content. For those vapers who want to quit nicotine altogether or simply enjoy vaping for the flavors, these devices offer a convenient and hassle-free experience. To this extent, we will explore the features, benefits, and reasons why zero-nicotine disposable vapes have become increasingly popular among vaping enthusiasts. So, sit back, relax, and discover a nicotine-free vaping experience like never before!
Ready to dive into the fascinating world of Delta 9 carts? Buckle up and get ready for an exhilarating ride as we unveil the secrets behind these mind-blowing creations. Sit back, relax, and let me spill the beans on how these bad boys are made. A team of dedicated artisans with a passion for crafting the perfect vaping experience. They take the finest ingredients and blend them with precision, creating a symphony of flavors that will have your taste buds doing the happy dance. These maestros carefully select strains known for their exceptional Delta 8 Carts and Delta 9 THC content, ensuring a potent and unforgettable journey. Now, let’s talk process, Each Delta 9 THC cart is intuitive through a combination of science and artistry. The first step is extracting the purest Delta 9 THC oil from carefully cultivated cannabis plants. It’s like capturing lightning in a bottle, my friends! This oil is then meticulously refined and purified, removing any impurities to guarantee a smooth and clean hit every time.
Virgin Atlantic Clubhouse at JFK Airport is a luxurious oasis for travelers seeking comfort and relaxation before their flights. Located in Terminal 4, the Clubhouse offers an exclusive experience that epitomizes the airline’s commitment to providing top-notch service to its passengers.
Upon entering the Virgin Atlantic Clubhouse JFK, guests are greeted with a contemporary and stylish ambiance, exuding the airline’s signature brand image. The well-designed space features various seating areas, including plush sofas and private workstations, catering to different preferences and needs of the passengers.
Are you looking for escort service in Zirakpur ? Book Meribillo Best and Cheap escorts service in Zirakpur. We Provide Female Zirakpur escorts working 24/7.
Ludhiana call girls
Mohali escort service
Chandigarh call girls
call girls in chandigarh
Ludhiana escort service
Zirakpur escort service
Mohali escort service near me
mohali call girls
call girl in chandigarh
While the game may seem simple, it is essential to understand that Satta King is illegal in many parts of India due to its exploitative nature and potential for fostering addiction. Despite its illegality, the game persists in some regions due to the allure of quick money and the lack of legal betting alternatives. Moreover, the secretive and underground nature of the game makes it challenging for authorities to completely eradicate.
Software testing is a critical process in the development of software applications to identify and rectify defects, bugs, or errors in the software. The primary objective of software testing is to ensure that the software meets its intended requirements, functions correctly, and delivers a satisfactory user experience. By systematically examining the software, testers can help enhance its quality, reliability, and performance.
Key aspects of software testing include:
Types of Testing:
Unit Testing: Testing individual components or units of code to ensure they function correctly in isolation.
Integration Testing: Verifying that different units or modules of the software work together smoothly.
System Testing: Testing the entire software system as a whole to ensure all components integrate correctly.
Acceptance Testing: Ensuring that the software meets the user’s requirements and is ready for deployment.
Performance Testing: Assessing the software’s responsiveness and stability under various conditions.
Security Testing: Evaluating the software’s resistance to unauthorized access or malicious attacks.
It seems like cpprep4005 answers might be a course code or a reference to a specific topic related to preparing to work in real estate trust accounts. While I don’t have access to specific course materials, I can provide general guidance and information on what working with real estate trust accounts might entail and the skills required to excel in this field.
Chill out with Flum Gio Litchi Ice, a tantalizing e-liquid blend. This exquisite flavor infuses the exotic sweetness of litchi fruit with a refreshing icy twist. Delight your senses with each inhale as the luscious litchi flavor dances on your taste buds, perfectly complemented by the invigorating ice undertones. Escape to a tropical paradise with Flum Gio Litchi Ice today.
With a heart full of wanderlust and a yearning for new horizons, I had the privilege of interacting with the warm and attentive staff at Singapore Airlines Brisbane Office. From the moment I stepped into their office, I was greeted with a smile and a genuine willingness to assist. Their expert guidance and personalized approach made every step of my travel experience seamless, from booking my flights to resolving any inquiries I had. Singapore Airlines Brisbane Office truly goes above and beyond to ensure that every traveler’s journey is nothing short of extraordinary.
The office is set up to answer questions from clients, deal with issues, and offer advice on travel-related problems. Garuda Airlines Toronto Office entire staff promises to provide timely and dependable help with all requests, including flight changes, cancellations, baggage questions, and special requests. The office may handle group bookings for passengers planning group journeys, such as business or family vacations, ensuring a simple and organised travel experience for everyone involved.
https://www.justcol.com/address/garuda-airlines-toronto-office/
Vidalista 20 medicine, contributes to manage the problem of erectile dysfunction. Vidalista gives rapid improvement in the performance by treating erectile failure. It is necessary to take the vidalista medicine with water before having the food as heavy meal reduces the effect of the medicine.
who don’t like an Affordable online task Assistance? Our specialists work very reckless, so they can quality your paper in as little as 2 hours. We always contain extremely pertinent and you can get check plagiarism report and high-quality report information in each task. In adding, every tradition task is written from cut to avoid stealing. With easy information about check plagiarism online Scholars will even get a correct my grammar with each thesis.
swiss airlines flight change fee ensures flexibility and convenience, allowing you to make necessary adjustments without unnecessary hassle. Stay prepared and informed to manage your travel arrangements efficiently with Swiss Airlines.
Thanks Vibha is a girl that will give relief to your erect penis. My pussy is tight, warm and full of sexual moisture.
HLTAAP003 is a unit that focuses on understanding human anatomy and physiology. It is essential for individuals working in the health and medical fields to have a comprehensive knowledge of the human body’s structure and its functions. This assessment aims to evaluate the learners’ understanding of key concepts related to human anatomy and physiology.
Hii my name is John Harrison. Facing HP printer problems? Get expert solutions here! Our comprehensive troubleshooting guide addresses common issues like paper jams, connectivity errors, and print quality problems. Let us help you resolve your printer headaches efficiently and get back to smooth printing in no time
This is really a Awesome blog have you written.I really enjoyed reading your post.I will be look forward to read your next post.
It is very informative to have this information shared with me. WebPays is a leading high-risk forex merchant account provider in Europe, offering the best payment services at the lowest MDR. To get quick approval, Appy Now.
Welcome to our Male to Male Massage Centers, where tranquility meets indulgence, and stress fades away. Our dedicated and experienced team of male masseurs is here to cater exclusively to men, providing a soothing haven for relaxation, rejuvenation, and holistic healing.
Welcome to our premier Home Service Massage in Delhi, where tranquility and wellness come knocking at your door. Say goodbye to traffic woes and the stress of commuting because we bring the indulgent world of massage therapy right to your doorstep.
“Indulge in rejuvenating call girls in Uttam Nagar. Our skilled and experienced massage therapists offer a range of therapeutic massages that aim to relax your muscles, reduce stress, and promote overall well-being. Whether you prefer a Swedish massage for gentle relaxation or a deep tissue massage to target specific areas of tension, our tranquil and hygienic spa environment ensures you’ll leave feeling refreshed and revitalized. Treat yourself to a blissful escape and let the soothing touch of our experts melt away your worries.”
The main procedure of the Wavlink AC1200 repeater is to repeat the WiFi signals in a new area that comes from the existing router. Consider the lower information to do the configuration process of the Wavlink AC1200 repeater.
To begin with, place the Wavlink AC1200 repeater power plug in the socket. After that, take the WiFi gadget and go to the WiFi settings of your WiFi device. From there pick the Wavlink repeater default network and connect the computer to it. Then open the browser and type in wifi.wavlink.com in the address bar. Later, tap on the setup wizard option and then pick the WiFi network that you want to boost. Now, fill in the details such as password, and hit on the connect icon. By doing this, the Wavlink ac1200 repeater setup process is complete now.
In any case, if you are facing trouble then try to place the repeater closer to the router, improve the software of the Wavlink AC1200 repeater from time to time, and don’t settle the repeater near to electronic appliances. By following these solution tips you can fix any kind of Wavlink repeater issue.
do your assignment projects given by the teachers need to be structured properly, and that calls for professional assistance. As a result, students do research and study all day and all night, and the majority of students still do not achieve A+ ratings on their assignments. As a result, they prefer to look for pay someone to do my assignment service online that offers assistance from a group of knowledgeable writers with PhDs or bachelor’s degrees that are competent in every subject related to nursing assignments.
Launchpad CPSB Apk aims to bridge the gap between traditional teaching methods and modern technology, making learning more accessible and engaging for students of all ages. https://androidmu.com/launchpad-cpsb-apk/
A influência positiva de Vênus em seu signo traz boas energias para evitar atritos no amor e perdas financeiras. Terá de controlar as questões profissionais e entender que precisa ser paciente.
One of the most popular escort services is executive female service for businessmen and executives who come to this famous city for business transactions. Radhika Apte ki Chudai ||
CorporatesOffice is a comprehensive online platform that offers extensive information about the American Airlines Corporate Office. This office serves as the central hub for American Airlines’ corporate operations, providing valuable resources and support for corporate clients and stakeholders. At CorporatesOffice, users can access a wide range of information related to the American Airlines Corporate Office, including contact details, executive profiles, company news, and updates on corporate policies and initiatives. The platform ensures that corporate clients have easy access to the necessary information they need to engage with American Airlines for business-related matters. Whether it’s exploring partnership opportunities, seeking corporate travel arrangements, or addressing specific inquiries, CorporatesOffice serves as a reliable source of information for navigating the corporate landscape of American Airlines.
CorporateAirlinesOffices is a comprehensive online platform that offers detailed information about Turkish Airlines Atlanta Office in Georgia, USA. This office serves as a central hub for corporate clients and travelers seeking assistance or information regarding Turkish Airlines’ services, bookings, reservations, and other inquiries in Atlanta, Georgia. CorporateAirlinesOffices provides a user-friendly interface that ensures easy access to up-to-date information about Turkish Airlines’ Atlanta office. Whether you need assistance with corporate travel arrangements, group bookings, or specific inquiries related to your travel needs, CorporateAirlinesOffices serves as a reliable resource to help you navigate your Turkish Airlines experience effectively in Atlanta, Georgia, USA.
Airlines Offices is a comprehensive online platform that provides detailed information about Spirit Airlines Orlando International Airport Terminal (MCO). This specific terminal, located at Orlando International Airport in Florida, serves as a key point of contact for passengers seeking assistance or information regarding Spirit Airlines’ flights, services, and amenities at MCO. AirlinesOffices offers a user-friendly interface where travelers can find up-to-date details about the Spirit Airlines Orlando International Airport Terminal, including terminal facilities, check-in procedures, baggage services, and other relevant information. With the help of AirlinesOffices, passengers can navigate their journey through MCO smoothly, whether it’s checking flight status, locating services, or obtaining general airport information related to Spirit Airlines.
Learn about the specific guidelines for pet carriers, size restrictions, and health requirements. With philippine airlines pet in cabin options, you can enjoy a hassle-free journey, knowing your pet is safely by your side throughout the flight.
Hii,
This is great and awesome post for me. i loved to read your blog. it’s really-really amazing. thanks for inspired me by your blog. https://adarsheuphoria.co/
Excellent .. Amazing .. I’ll bookmark your blog and take the feeds also…I’m happy to find so many useful info here in the post, we need work out more techniques in this regard, thanks for sharing.
Discover a hidden gem that will delight wildlife enthusiasts and outdoor adventurers alike – “Wildlife Ranches.” This website boasts a seamless and visually appealing design, ensuring a delightful browsing experience from the very beginning.Ranches
zxxxDiscover a hidden gem that will delight wildlife enthusiasts and outdoor adventurers alike – “Wildlife Ranches.” This website boasts a seamless and visually appealing design, ensuring a delightful browsing experience from the very beginning.Ranches
I’m happy to find so many useful info here in the post. Kate Upton Astros Sweater
Oh my goodness! an incredible article dude. Thanks Nonetheless I am experiencing subject with ur rss . 메이저사이트
Thanks for the information you have provided us
cheap flight from newyork
law Assignment Help in Canada is a valuable support system for students pursuing legal studies. With expert guidance and comprehensive solutions, students can navigate complex legal concepts, excel in assignments, and develop lifelong skills for a successful legal career.
Step into the captivating world of “WildlifeRanches.com,” an exclusive sanctuary for wildlife enthusiasts and daring explorers. Immerse yourself in its mesmerizing design, explore invaluable content, and master expert hunting tips. Whether you’re captivated by wildlife ranchesor seeking adrenaline-pumping experiences, we cater to all your interests. Embark on an unforgettable journey and embrace the untamed wonders of the wild today at “WildlifeRanches.com,”
With eva seat selection, personalize your journey for optimal comfort and convenience. Enjoy a hassle-free reservation process, ensuring you have the best seat for a pleasant flight. Elevate your travel with EVA Air.
Renowned for its invigorating properties, the best white kratom strain is a standout among its counterparts. With a unique alkaloid composition, it offers a harmonious blend of energy and mental clarity. Best white kratom strain, often sourced from Southeast Asia, is favored for its potential to enhance focus, boost mood, and provide a natural energy lift.
In the center of Southern California’s entertainment and attraction hub, guests can easily get to their wonderful location and start their enchanted trip. The Nearest Airport To Disneyland offers a variety of domestic flights, making it a popular option for those looking for a simple way to visit Disneyland.
I have enjoyed reading your blog. As a fellow writer and Kindle publishing enthusiast, I would like to first thank you for the sheer volume of useful resources you have compiled for authors in your blog and across the web. I’m also working on the blog I hope you like my Handmade Jewelry blogs.
Fantastic Blog!! Thank you to Admin for providing the above list. I browsed over several of your Blog’s pages. Your blog is fantastic. Continue to share such wonderful stories.
Aeromar Teléfono
So nice article, i found it very explanatory and informative, keep sharing the best content. Join the league of trendsetters and get noticed wherever you go – wear our timeless leather jacket and be unforgettable Women Fur Coats
Risk Management Assignment Help equips students with the knowledge and skills to analyze, assess, and mitigate potential threats or uncertainties that can impact the success of projects, businesses, or endeavors. Students delve into techniques like risk identification, risk assessment matrices, and risk response planning. Such assignments not only enhance students’ understanding of risk management principles but also prepare them for real-world scenarios where informed decision-making and proactive planning are essential to navigate the complex landscape of uncertainties effectively.
Nearest Airport To Richmond
Se Quiere Saber Que es Aeromar Teléfono Para Que Usted Se encuentre en el Lugar Correcto Donde Puede Encontrar Información Actualizada sobre Aeromar Ailrines Teléfono
Cheap Flights From Houston to Los Angeles
We really appreciate you sharing this incredibly useful information. Explore Carpets Direct for the finest collection of Carpets in London. Quality styles at unbeatable prices. Enhance your space with our luxury pieces. Shop now.
In Singapore’s fast-paced academic environment, students often find themselves overwhelmed by demanding assignments. Embracing online assignment help in Singapore can be a game-changer. These services connect students with experienced experts who offer personalized assistance in tackling various assignments. With just a few clicks, students can access guidance on complex topics, ensuring assignments are well-researched and structured. Online assignment help also offers the flexibility of 24/7 accessibility, accommodating busy schedules. From essays to presentations, these services not only improve grades but also foster a deeper understanding of subjects. By leveraging online assignment help, students can confidently navigate their academic journey, manage workloads, and enhance their overall academic performance, all while gaining valuable insights from seasoned professionals.
Struggling with Supply Chain Management assignments? We’ve got you covered! Our professional team provides top-notch Supply Chain Management Assignment Help tailored to your needs. Our experts ensure in-depth understanding, timely delivery, and original solutions. Contact us now our professional support team for reliable, affordable, and plagiarism-free assignment help.
I am glad to read this post, it’s an impressive piece. I am always searching for quality posts and articles and this is what I found here, I hope you will be adding more in future. Embrace the rebel within and conquer the ordinary – our leather jackets are your key to standing out in the crowd Blue Biker Jacket
In essence, it’s a journey that unveils the potential results of a chosen path, often tied to decisions or choices that one must make
This was really amazing, keep up the great learning. If you don’t know, what is Twitch? Then don’t worry, Twitch is a popular online platform primarily used for live streaming and watching live broadcasts of video games, For complete info about this, I would suggest you can read one blog about Twitch Adblock, where you’ll learn everything about twitch.
finance assignment help online is plenty to study if one wants to become a nursing expert, making it a very technically challenging and complicated subject. Being a nursing practitioner needs both academic and practical expertise, making it a very demanding field. It’s crucial for nursing students to complete a variety of coursework and case studies that simulate real-world conditions. Therefore, in order to properly resolve challenging case studies, it is crucial to possess solid subject understanding. The authors at pay someone to do my assignment services are highly trained and have decades of expertise. They excel at managing nursing tasks across a variety of nursing specialties, such as nursing for adults, kids, learning difficulties, and nursing for emotional well-being.
That is nice article from you , this is informative stuff . Hope more articles from you . I also want to share some information about Best Digital marketing service in india
Thank you very much.
infotechinn offers news and updates on the most recent technology, creative startups, marketing strategies, and fashion technology
Seeking good quality assignment help in USA? Look no further! Our team of experts who have lots of experience in the subjects is here to help you in achieving your academic goals. Whether it’s a complex or difficult assignment, we have got the winning formula. You will get stress-free mind, remarkable grades, and time for your passions. Our services are trusted and we make sure about confidentiality and originality. Therefore, don’t miss out on this opportunity to excel! Let us be your help providers to help you in your success. Say goodbye to your academic stress and achieve the top grades with our unstoppable assignment help.
That is nice article from you , this is informative stuff . Hope more articles from you . I also want to share some information about Best Herbal products in India
frontier sfo terminal
Delhi is a city of joy and enjoyment where the source of such kinds of enjoyment and happiness are immense and most popular as well. It is the reason several thousands of people from around the world would gather there and most probably you will never be able to share out some of the best opportunities that you must share and it is the reason several kinds of entertainment and flavors are richly filled with some of the most pleasing activities. Escorts in Delhi The main reason behind such kinds of interesting and entertaining elements mainly include of sexual pleasure offered by none other than the richly flavored independent delhi escort service Uzbek American Escorts Girls in Delhi and service which has become so much enjoyable and pleasing as well.
I have read your blog. Your blog is very interesting.
If you are struggling with your Assignment Writing then do not worry,
Get Assignment Help from Instant Assignment Help.
Thank you sharing such a useful information. I’m Writer by profession and i found that today’s student are very curious about their assignment. I recommend Instantassignment help,this website provide best assignment writing service . Instant Assignment Help is an online platform that offers academic writing services to students worldwide. One of the key roles within their team is that of an assignment writer. An assignment writer is a professional who specialises in crafting high-quality and well-researched academic papers on various subjects and topics.
Expert Project Management Assignment Help for precise planning & execution. Achieve academic success with our guidance. Get assistance today!
Ang Kalusugan Blog ay isang kapaki-pakinabang na website para sa impormasyon sa kalusugan. Mayroon itong madaling gamiting impormasyon tungkol sa pananatiling malusog at pag-aayos ng mga problema sa kalusugan. Maaari kang makakuha ng kaalaman tungkol sa pagkain, ehersisyo, at kung paano maging maganda ang pakiramdam tungkol sa iyong sarili. Ang mga taong naghahanap ng mga tip sa mabuting kalusugan tulad ng website na ito dahil ito ay simple at tama.
Are you stuck and looking for the best leadership research topics to ace your grades? If your answer is yes, then you have come to the right place. This blog by assignment help has covered interesting Intriguing Leadership Research Topics for you and also provided some tips on how you can write a research paper that can help you increase your grades.
You there, this is really good post here. Thanks for taking the time to post such valuable information. Quality content is what always gets the visitors coming.
Have you ever played on varioussweet.games ? This site of games in this game has a lot of incredible things that the real games does not have. For example, coating girls, cooking and a kitchen with lots of delicious food. You can even use all the food to feed the characters. In short, you can interact with almost everything in this site of it. And it’s easy to find things to interact with.
Are you searching for the best assignment help for BPP University? Look no further! our dedicated team of experts is here to provide you with graded quality assignment help in achieving your academic success. Whether you need help with business, management, accounting, or any other subject, we will provide help and our service makes sure of timely submission, good quality, original content and in-depth research. And trust us that, by selecting us as your help provider you will be able to say goodbye to academic stress and hello to your outstanding grades as your academic excellence is our priority.
Greetings to all fellow aficionados of delectable delights! I am Anika Shree, an avid lover of the culinary arts in all their glory. It is with genuine excitement that I take on the role of your guide today. Join me on an escapade to unearth the best view cafe in Dehradun – Narcoz Food Cartel. This cafe stands as a true embodiment of excellence. The ambiance itself is a masterpiece, reflecting the sublime natural beauty that envelopes us. An invitation to dine here is an invitation to partake in pure enchantment.
Thanks for your blog
James Joyce Assignment Expert | Helping Students Excel
Greetings! I’m James Joyce, a dedicated professional offering top-notch academic assistance to students across the USA. With a passion for education and a wealth of expertise, I am committed to helping students achieve their academic goals.
About Me: As an assignment expert, I specialize in providing comprehensive “Computer Network Assignment Help” services that cater to the unique needs of students.I possess a deep understanding of the challenges that students often face in today’s fast-paced educational environment.
I am sincerely grateful for the exceptional assignment help services provided. The support extended went far beyond my expectations. The dedication and expertise displayed by the team were truly remarkable. Not only did they deliver a meticulously researched and well-structured assignment, but they also ensured that it was tailored to my specific requirements. Their commitment to quality and timeliness were evident throughout the process. This assistance has not only eased my academic journey but also enhanced my understanding of the subject matter. I wholeheartedly appreciate the professionalism, reliability, and excellence exhibited by the assignment help services. Thank you for being a beacon of academic support!
Stuck on outlook stuck on loading profile‘? Fret not; you are in the right place. Hello, I’m your Outlook loading issue troubleshooter. My name is Liam, and let me take the frustration away. With my expertise, I can troubleshoot this snag, ensuring your Outlook runs smoothly. Connect with me or my tech support team 24/7 for immediate solutions.
Are you stuck and looking for the best leadership research topics to ace your grades? If your answer is yes, then you have come to the right place. This blog by assignment help has covered interesting Intriguing Leadership Research Topics for you and also provided some tips on how you can write a research paper that can help you increase your grades.
Dear immortals, I need some wow gold inspiration to create.
The LOT Polish Airlines Warsaw office has played an important part in the growth of Poland’s aviation industry. It has aided in the establishment of Warsaw Chopin Airport as a major European hub, connecting travelers from all over the world. LOT’s dedication to safety, innovation, and customer happiness has won it a superb reputation in the aviation industry.
Hire experts to get the best Excel assignment help at 30% Off. Get instant help 24/7 at an affordable price. Choose us to score A+ on your assignments.
Hire experts to get the best Excel assignment help at 30% Off. Get instant help 24/7 at an affordable price. Choose us to score A+ on your assignments.
Excel Homework Help
Hulu ad blocker is a browser extension that helps block advertisements while streaming content on the platform, it prevents ads from playing during TV shows, movies, and series that allow you to watch your favorite programs and shows without any disruptions.
Look no further than a YouTube watch party! Enjoy YouTube with your friends, or loved ones, no matter where you are. Use live chats, videos, and calls to talk and have fun together. It’s like having your own showtime parties, even if you’re far away!
Optimistic Authors distributes the best if you opt for pay someone to do my online philosophy class . philosophy projects are an essential part of researchers for commercial, law, and medicinal scholars. These preps are real-life imitations that need in-depth investigate and examination. Scholars must classify the problematic, collect, analyse, and deliver references. However, these projects can be fairly inefficient and stimulating. This is anywhere the philosophy online assignment help specialists originate in.
Great Information. Also, read this blog on Tenant to Tenant Migration. It will help you migrate mailboxes from one tenant to another in Microsoft 365.
Elevate your dental practice’s online presence with SDAD Technology, the premier Dental SEO Agency. With a proven track record of success, we specialize in boosting your practice’s visibility on search engines, driving more patients to your doors. Our dedicated team of SEO experts understands the unique challenges of the dental industry, tailoring strategies to your specific needs.
At SDAD Technology, we employ cutting-edge SEO techniques, keyword optimization, and local search strategies to ensure your practice shines in your community. Experience increased website traffic, higher rankings, and a steady stream of new patients. Trust SDAD Technology to take your dental practice to new heights in the digital age.
This is a very informative content, I appreciate that author has taken time for such a unique content. Discover the allure of confidence and sophistication – grab your leather jacket and make a bold fashion statement today! Men biker jacket
If you’re in Cambridge or elsewhere, we can help. We are a reputable Cambridge female escort service with a team of skilled and knowledgeable escorts who can properly satiate your entire spectrum of sexual desires in your style. One of our beautiful girls can help you get the fulfillment you seek. bassse You don’t have to give in to your impulses if you reside in Cambridge. We can offer you a range of excellent services to satisfy your sexual desires.
Welcome to LOT Polish Airlines Newark Terminal – your door to a universe of movement and experience. LOT Polish Airlines is situated at Terminal An at Newark Liberty International Airport (EWR). Voyagers flying with LOT Polish Airlines can track down their registration, takeoff entryways, and different administrations inside Terminal A. This terminal offers a scope of conveniences and offices to guarantee a smooth and charming travel insight for travelers flying with LOT Polish Airlines.
Field Engineer – A Global On-demand Freelance Marketplace. Field Engineer, headquartered in New York, United States and spread its operations & services globally with its branch office in Hyderabad. FE is a powerful platform for businesses and talent who want to be free of old-school recruitment hurdles. We streamline slow and costly talent and job search, interviewing, vetting, hiring and paying. FE frees the ambition to succeed. FE is proving to be an indispensable global marketplace – Types of Desktop Virtualization
Exter Networks, a Global Managed IT Service Provider is not new to meeting the challenges of Managed Technology Services such as Network Operations Center Services (NOC), Security Operations Center Services (SOC) etc. Since 2001 we’ve exceeded expectations using our Full Technology Lifecycle Support Model that provides end-to-end solutions featuring Design, Deployment and 24/7/365 support – SQL Injection Attack
¡Este artículo está realmente bien escrito! El escritor explica ideas complejas de forma sencilla, haciéndolas fáciles de entender. El artículo está muy bien organizado y me mantuvo interesado todo el tiempo. ¡Aprendí mucho de eso. Si buscas escorts en Argentina puedes consultar esto:- escorts Mendoza
Optimistic Authors distributes the best if you opt for online economics assignment help US. Economics projects are an essential part of researchers for commercial, law, and academics scholars. These preps are real-life imitations that need in-depth investigate and examination. Scholars must classify the problematic, collect, analyse, and deliver references. However, these projects can be fairly inefficient and stimulating. This is anywhere the Economics online assignment help specialists originate in.
Optimistic Authors distributes the best if you opt for pay someone to do my economics assignment . Economics projects are an essential part of researchers for commercial, law, and academics scholars. These preps are real-life imitations that need in-depth investigate and examination. Scholars must classify the problematic, collect, analyse, and deliver references. However, these projects can be fairly inefficient and stimulating. This is anywhere the Economics online assignment help specialists originate in.
Totally! Your questions are great and show a real desire to learn. It is inspiring to see someone actively searching for information and participating in lively discussions. This is an excellent opportunity to show off your knowledge and passion. You can gain a better understanding of your life and yourself by asking questions. Your curiosity will prove to be an invaluable asset in your journey. Never stop exploring and using your curiosity. Every question can lead you to amazing insights and discoveries.
Best Training Institute in Pune
India has one of the highest household savings rates in the world; the Indian stock market is still in its. Here is the List of Top 10 Mobile Trading Apps in India. You must check out infancy fundamentals about the app and invest with the Best Trading App in India
Are you in search of someone who will help you, and you can pay someone to take my online class for me? Then nobody, believe me, nobody, can assist you the way the experts of assignment help agencies can. So, grab the best deal in the USA’s online academic assistance services.
The engineering assignment help agencies can help you through getting guidance. Depending on the accurate reviews posted by the clients, the students have come to one decision for sure. Students ask for top notch help with engineering assignment help which is attainable at a very reasonable rate.
I found it worth reading. I just want to ask you to write more about Best Jewelry in Pakistan
Very interesting blog. Your explanations were clear and easy to understand, and your attention to detail was greatly appreciated. Keep up the fantastic work, and I can’t wait to read more of your insightful posts! If You Want any assessment help Visit out Site AssesmentHelp.com
We provide finance assignment help to students who need assistance with completing their academic assignments. The goal of assignment help is to provide students with support and guidance throughout the assignment writing process, to help them improve their writing skills, and to achieve academic success.
And for those who yearn for the enchantment of Midnight delivery in dubai , we guarantee that celebrations stretch far beyond the chime of midnight. Explore the zenith of online gifting excellence with us, where your expressions of love and care transcend the ordinary, crafting cherished memories that etch themselves into your heart for a lifetime.
I appreciate your fantastic blog.
Hello! I’m Benjamin, a travel writer who is constantly looking for unusual encounters. Examining LOT Polish Airlines at Newark Liberty International Airport (EWR) is what we’ll do today. EWR airport’s Lot Polish Airlines EWR Terminal is located in terminal B. The Lot Polish Airlines EWR Terminal is a stylish and welcoming area that skillfully combines comfort and aesthetics. My journey began quickly thanks to quick check-in alternatives including self-service kiosks. Before your trip, unwind in the LOT Business Lounge’s cosy retreat with plush seating, delectable refreshments, and interesting drinks. Quick boarding and a welcoming cabin crew ensured a relaxing trip with delectable meals and entertainment options. My expectations were met by LOT Polish Airlines, making EWR Terminal a top choice for travellers of all levels.
Thanks for the great post, I really enjoyed it and appreciate it. My job is about electronic components. If you are looking for some rare electronic components, maybe our website has the model you are looking for in stock.
This blog was very nicely formatted; it maintained a flow from the first word to the last. Step into style and embrace your edgy side with our premium leather jacket – a must-have for every fashion-forward individual! Blue Biker Jacket
If you’re a student in Malaysia, you might be facing the challenge of juggling multiple assignments, exams, and coursework. The pressure can be overwhelming, and at times, you may find yourself in need of some assistance. That’s where assignment help services come into play. In this Discussion, we’ll explore the options available to you and how to find the best Assignment Help in Malaysia that not only assists you academically but also ranks well on Google.
Thanks For Reading This!
Given the incredible amount of information in this post,
Michael Jackson Jacket Colelction Outfits
Thanks for sharing this valuable information with me. I am using Top Cisco 9200 nowadays as you know the Cisco Catalyst 9200 switches are made for the modern world as networking. We have affordable cisco catalyst products so check us out now.
Thanks for sharing this with so much of detailed information, its much more to learn from your article. Keep sharing such good stuff. Feel the luxury, wear the attitude – our handcrafted leather jackets are here to redefine elegance and prestige leather jacket for women
I found the post to be highly good. The shared information are greatly appreciated
Regards
Chauffeur service in Dubai
The post was truly enjoyable. I value your contribution and the information you shared.
Regards
Explore Dubai Tour packages
Thanks for sharing superb informations. Your web site is very cool. I’m impressed by the details that you have on this blog. Capture the essence of adventure with our leather jackets – conquer new horizons in style red puffer jacket
Your post is both informative and engaging. I’ll definitely bookmark this page for future reference. Thank you for sharing this post New York State Divorce Laws Marital Property
Traveling with luggage can be stressful. The LOT Polish Airlines Warsaw office offers valuable assistance regarding baggage allowances, restrictions, and tracking services, making your journey hassle-free.
Our Assignment Help service is a market leader in providing high-quality assignment writing services. Our skilled writers and editors are dedicated to providing personalized, plagiarism-free projects that satisfy the highest academic standards. With years of industry experience, we have successfully assisted hundreds of students in achieving their academic goals. We have the skills to offer remarkable results with essay help, research papers, case studies, and dissertations. To get started, contact us today!
Hii,
This is great and awesome post for me. i loved to read your blog. it’s really-really amazing. thanks for inspired me by your blog. https://adarsheuphoria.co/
“Absolutely top-notch PPC services! This company in Jaipur delivers exceptional results. Their expertise in pay-per-click advertising is unmatched. They’ve significantly boosted our online presence and ROI. Highly recommended for anyone seeking the best PPC solutions in Jaipur!”
Best PPC Company In Jaipur
“Startup registration by the Indian Government offers a plethora of advantages. It provides legal recognition, access to various incentives, and fosters investor trust. This initiative not only simplifies business operations but also fuels innovation and job creation, contributing significantly to India’s economic growth and global competitiveness. A commendable step towards empowering entrepreneurs!”
Benefits of Startup Registration By the Indian Government
Gulab ji Chai Wala is a hidden gem! His chai is a delightful blend of flavors, with the perfect balance of spices and sweetness. Every sip is a warm, comforting hug for your taste buds. His welcoming smile and friendly service make the experience even more enjoyable. A must-visit for tea lovers! ☕🌹
Gulab ji chai Wala
“Pain Guru” is a true lifesaver! Their expertise in pain management is unmatched, offering invaluable insights and guidance for those suffering. With a wealth of knowledge and compassionate support, they empower individuals to conquer their pain and regain their quality of life. A trusted source of relief and wisdom.
Pain Guru
Having a vast range of escorts with various backgrounds, physical characteristics, and personality helps draw in a wider clientele. People have varied preferences, so offering a wide range of options with Escort Services in Gurgaon increases the likelihood that you can satisfy all of your clients’ needs.
Thanks for the information. I really Like this. Southwest MCO Terminal is a hive of activity where passengers board flights with the renowned Southwest Airlines for trips to distant locations. Passengers can enjoy a flawless and practical journey from this terminal, which serves as a doorway to adventure. The Southwest Terminal at MCO makes sure that the thrill of traveling starts long before passengers board their flights with its contemporary facilities, quick check-in and security processes, and a selection of restaurants and shopping options.
MBA Assignment Help has been a game-changer for me during my MBA journey. Their expert guidance and timely assistance have not only reduced my stress but also significantly improved my grades. Their customized solutions cater perfectly to my assignment needs, ensuring I meet my professor’s expectations. It’s a wise investment in academic success, and I highly recommend their services to fellow MBA students. Thanks to them, I can focus on gaining a deeper understanding of business administration without worrying about assignment deadlines and quality.
This provides an excellent chance to investigate this website, and I’m thrilled to have discovered it. I’m grateful for the opportunity you’ve given us to take full advantage of this offer Outdoor accessories in USA
Generally, CBSE class 12 exams are conducted during the month of March. Before that, both teachers and students need to complete the class 12 syllabus. After the completion of the syllabus, you can take the help of CBSE Sample Paper Class 12 as it provides more questions. This can help you to understand the weak points and can accordingly work upon it.
The linksys re6400 setup is a hassle-free way to extend your WiFi coverage. With the Linksys RE6400 extender, you can eliminate dead zones and enjoy a stronger connection in various corners of your space.
Great post having a lot of information!! Really it’s a very helpful guide. Thank you for sharing.Order
Statistics Homework Help from qualified experts.
Apkchowa is a apk library site to stored apk file from playstore. So Download App and games directy without google playstore
I recently visited Kashmir and I had a wonderful experience with kcs
Srinagar Cab Service’s commitment to a smooth ride is evident in their well-maintained cars. I enjoyed a comfortable and stress-free journey. Choose their cab service in Srinagar for a pleasant travel experience.
The Qatar Airways New York Office is more than simply a place to book flights; it is also a hub for travel information. The company employs highly experienced experts that are familiar with every aspect of air travel. Whether you’re seeking information on flight schedules, fare alternatives, or need assistance with reserving a ticket, the knowledgeable personnel at Qatar Airways are here to provide individualized advice and recommendations customized to your travel needs.
Calafia Airlines Teléfono
Assignment Help services in Canada are a lifeline for students. They provide crucial support, helping students navigate challenging subjects and manage academic pressures. With expert guidance, timely assistance, and valuable insights, these services empower students to excel in their studies and maintain a healthy work-life balance. They are an indispensable part of the Canadian student experience, contributing to academic success and personal growth.
I am a Certified Internet Research Professional with experience managing Internet research and a broad understanding of Internet zaba people search, job boards, research associations, groups, and forums. I conducted online research on several websites and kept track of all materials. This certification taught me all of these skills. I must say this is the best training course for researchers.
Winter training is a great way to enhance your skills and knowledge, and gain a competitive edge in the job market. If you’re looking for [url=https://www.cetpainfotech.com/winter-training]winter training in Noida[/url] or Delhi, there are a number of excellent institutes to choose from.
Great Information! Thank you for sharing such an informative post.
If you’re interested in learning Technology, I recommend checking out the winter training in Noida
More than just a site to make airline reservations, the Qatar Airways New York Office serves as a resource for travel information. The organization works with highly qualified professionals that are conversant with every facet of air travel. The educated staff at Qatar Airways is here to provide individualized advice and recommendations tailored to your travel needs, whether you’re looking for information on flight schedules, fare options, or require assistance with reserving a ticket.
I found the most beautiful and fascinating one.
Excellent blog! Such clever work and exposure! Keep up the very good work.
Hello, I’m, a dedicated academic writer from London, UK. As a proud member of Dissertation Help, I’m committed to empowering students on their educational journey. With expertise in various subjects, I provide top-notch assignment assistance to help students excel in their studies. Trust in my experience and dedication for academic excellence in London, UK’s dynamic educational landscape.
Your blogs are informative and useful keep sharing such good knowledge through your blogs.
Also read:- Artificial Intelligence Training in Noida
Dear immortals, I need some wow gold inspiration to create.
So nice article, i found it very explanatory and informative, keep sharing the best content. Join the league of trendsetters and get noticed wherever you go – wear our timeless leather jacket and be unforgettable Purple Leather Jacket
Most likely, you’ll use a service’s mobile app or website to book Call Girls In Aerocity. You can browse women who are accessible for you to take out using these media. You can view the women’s photos, interests, and typically their rate while browsing.
Do you find yourself unable to keep up with your workload? Seeking assignment help from others with academic assignments is a smart move that may lighten your load and provide you with more spare time. The expert writers can complete your papers on schedule and at the highest standard. Based on the specifics of each student’s request “write my assignment” experts then have subject-matter experts respond to their homework questions. They know precisely what areas need to be in your essays, dissertations, case studies, and reports in arrange for you to get a good grade. Do not rush anything; instead, begin working immediately to raise your grades.
Welcome to contact a professional Aerosol Foundation Spray factory.
Facing a problem with the Wavlink AX1800 setup? Not to worry follow the detailed instructions step by step to connect your extender to your home or office wireless network with ease. The WL-WN573HX1 is compatible with the most recent wireless standards, such as 80 MHZ and 802.11ax (Wi-Fi 6). You can simultaneously stream on two different bands thanks to the device.
Thanks for sharing superb informations. Your web site is very cool. I’m impressed by the details that you have on this blog. Capture the essence of adventure with our leather jackets – conquer new horizons in style. brown leather jacket womens
law assignment help services in Canada play a crucial role in enhancing students’ educational experiences. They provide expert guidance, helping students grasp complex legal concepts and improve their academic performance. By lightening the academic load, these services enable students to pursue a more balanced life, focusing on personal growth and extracurricular activities. This holistic approach fosters better education and enriches student life.
If the demands of online classes are overwhelming and time seems to slip away, consider the option: “Take my online class for me.” The modern educational landscape has embraced flexibility through virtual learning, but juggling multiple courses, assignments, and commitments can be challenging. Outsourcing your online class can provide a practical solution.
Great article with excellent idea!Thank you for such a valuable article. I really appreciate for this great information..
Soloop App
For Peaceful time in dal lake srinagar you can do shikara ride in dal lake , you can do online bookings for shikara ride with us.
shikara ride in dal lake
You can improve the speed and coverage of your wireless network with the mywifiext.net setup. If you’re facing slow WiFi or weak connections, you can follow these instructions for manual configuration or use the WPS method for a quick setup. Additionally, we’ll cover performing a factory reset and updating the extender’s firmware.
Assignment Help Canada: Get professional online assignment help from experienced Canadian writers. We offer high-quality, custom-written assignments in a variety of subjects and academic levels. Guaranteed satisfaction!
Here are some other keywords that you can use in your description:
Canadian assignment help
Research paper writing help
Plagiarism-free work
24/7 customer support
We believe it was done by whoever finished it.
This Link
Great article! The insights shared here are both informative and thought-provoking.
When facing the daunting task of analyzing complex scenarios, students often turn to Case Study Help and Case Study Helpers to navigate the intricacies of their assignments. These invaluable services offer expert guidance in deciphering real-world problems, ensuring students grasp the core concepts and analytical methods required for effective case study analysis. Case Study Help provides a lifeline for those struggling with the research and structuring of their cases, while Case Study Helpers offer tailored assistance, turning complex situations into manageable learning opportunities. Together, they empower students to excel in their studies by offering support and insights critical for success in case study assignments.
The expedition begins in the quaint town of Pahalgam, from where you’ll be transported to the starting point of your rafting adventure in Pahalgam.
This is a very informative content, I appreciate that author has taken time for such a unique content. Discover the allure of confidence and sophistication – grab your leather jacket and make a bold fashion statement today! fear of god essentials hoodie
Struggling with your economics assignments? Our Economics Assignment Help service is your ultimate solution! We have a team of experienced economists and academic experts who specialize in various economics topics, from microeconomics to macroeconomics, econometrics, and more. No matter how complex your assignment is, we provide clear, concise, and well-researched solutions tailored to your requirements. Our dedicated team ensures timely delivery, allowing you to meet your deadlines with ease. With our expert guidance, you can grasp intricate economic concepts, enhance your understanding, and secure top grades. Don’t let economics assignments stress you out – let us handle them while you focus on your studies and future success!
Allegiant flight change policy
파워맨의 모든 제품은 100%수입산 제품입니다, 절대로 국내에서는 구하지 못하는 미국,유럽,캐나다,인도 등 나라에서 판매하는 제품만 취급합니다. 중국산 제품은 절대로 판매하지 않습니다.
Assignment Help Canada: Get professional assignment help from experienced Canadian writers. We offer high-quality, custom-written assignments in a variety of subjects and academic levels. Guaranteed satisfaction!
Here are some other keywords that you can use in your description:
Canadian assignment help
Research paper writing help
Plagiarism-free work
24/7 customer support
Awesome Blog !!! Thanks to Admin for sharing the above list. I visited many pages of your Blog. Really your Blog is Awesome. Keep Sharing such good Stories. Thanks.
GmailDisabledException is an exception in the Google API Client Library for Java, specifically related to Gmail API usage. When this exception is thrown, it indicates that the Gmail service is disabled for the user making the request. This could happen due to various reasons, such as the user’s Gmail account being suspended, restricted, or disabled by Google.
Read more: GmailDisabledException
Looking for reliable Assignment Help services? Our comprehensive guide covers everything you need to know about Assignment Help, from finding the right service to assistance for your academic needs. Many students face similar challenges during their academic journey. However, there’s a solution that help ease your academic burdens and pave the way for success assignment help services.
Each task, paying little heed to degree or intricacy, accepts our enthusiasm, commitment, ability, experience, and understanding to give fantastic results. GT Solution offers WordPress Web Development and Shopify Development Services. We’re responsible for building a website from concept to completion from the bottom up, fashioning everything from the home page to site layout and function such as Website design and development, Landing pages, and an e-Commerce Store, Design from mockups, converting PSD to WP, and expert in any custom design and development, Logo design, Icon, banner design and so on. So get in touch to Hire WordPress developer for your site and get 30 days free support after completion of site!
It was not first article by this author as I always found him as a talented author. Pink Ladies Jacket
Inforinn is the greatest blog website that provides latest updates related to technology, business, fashion. Here you can write for us + business
Thanks for the detailed information about this topic. I would love to see more such awesome blogs from you. Keep up the good work! Goku.Tu
The Italian bedroom is a masterpiece of design and style, capturing the essence of sophistication and elegance
Very insightful article, really appreciate it. As it help to assignment help online
Hello, author!
I just finished reading your marketing dissertation help article and couldn’t help but leave a comment to express my impression! Your ideas are not only informative but also extremely engaging. As a student struggling with my marketing thesis, coming across your article was like finding a treasure trove of valuable information.
Unlock the potential of mobile gaming with the Best Mobile Game Development Agency in Jaipur – Blockverse Infotech Solutions. Elevate your gaming experience with innovative, immersive, and captivating mobile games crafted by our expert team. Explore limitless possibilities in the world of gaming with our cutting-edge solutions and creative excellence.
hi are you looking for a furniture for your home, office then visit italian furniture
Someone essentially helps me make a serious article I want to mention. Is that what I frequently visit your website page? I was surprised by the analysis you did to make this actual submission hard to believe. It’s a fantastic work! 메이저놀이터사이트
Hello. I came to get a refund after seeing you visit my website. I’m looking for problems to improve the website. I think it’s okay to use a few ideas!! 신규토토사이트
“Hotline Miami Jackets have a distinct retro and edgy charm. Let’s explore what makes them so appealing. Are you a fan of this gaming-inspired fashion?
Share your thoughts on how these jackets bring gaming culture into everyday style and their role in the fashion landscape.”
If you do not have extra money and want to get hot girls, then try our Mukherjee Nagar Escorts for the best and most pleasurable experience for you. Our girls are mature, well-educated girls and come from upper-class families. So you don’t need to get into any fights, he has a good attitude. They are ready to make your evening very wonderful and beautiful and you can share your doubts with our girls.
Services that help effectively with writing Assignment Help Kuwait are welcome in Kuwait. Whenever you get stuck on a task, log on to the site to ensure they are creating quality courses that make difficult tasks easier by helping you solve them with minimal headaches.
Hello, I am Komal Sharma and I have been working as an escort for 5 years. We believe in empowering our clients to make serious decisions. that help them overcome isolation live an interesting life and move forward independently. Hot and sexy are two words that ring a bell along with the services provided by Chhatarpur Escorts.
If you already suffer from a dread of trigonometry, every wrong answer will shatter your self-assurance. That is why it is helpful to take advantage of online trigonometry courses and find a teacher online. Regular practice with the Take my online trigonometry class service-designed activities will help you hone your trigonometry skills and instil confidence in your abilities.
It appears that other students are experiencing the same difficulties in maths. Trigonometry homework, in particular, appears to significantly increase their burden. They get increasingly worried and anxious. Do you need trigonometry homework help because you are too stressed out to do it? Online class is the only hope for your worrying.
Get the latest New York Jets TV channel, news, videos, rosters, stats, schedule and NY Jets Gameday ways to watch. Enjoy all NFL season matches of Jets Game in any device from anywhere in Ultra HD Streaming quality.For more info visit our site:Watch Jets Football Game Live Online
This was an extremely wonderful post. Thanks for providing this info. Jack Torrance Jacket
In today’s fast-paced world, taking the time to select and send a Congratulations Cards shows that you genuinely care. It’s a small gesture that can make a big difference in someone’s day.
Moti Bagh Escorts is a popular group of girls who have been working for a long time. They are recognized for their grandeur, dazzling services, low-cost fees, amazing work, and imaginativeness of the job as well as services. Therefore there are many people with different types of interests who hire Moti Bagh Escorts for their personal services. These hot girls are educated and know the art of satisfying the needs of their customers.
Dr. J MD is a highly qualified physician, Dr. J has spent years in research on cosmetic and aesthetic medications. According to Dr. J MD BOTOX® has proven to be a versatile treatment option, catering to various cosmetic concerns.
My job as a manager is not a piece of cake. Handling a team of Noida call girls is not a simple task. First receiving hundreds of calls from morning to night and then closing the deals is quite a hectic work. Sometimes, especially on the weekend, the load of work sucks the air out of my lungs. But still, I am happy with my job. Why? Because my Noda escorts agency is considered the best agency. When customers say “Thanks” to me with a cherishing smile on their faces, I feel a heavy sense of pride. My profession might be objectionable, but still, it is all about love and care. Love seeking people come to me and my girls take care of them. What’s wrong with that? After all, we live life once. So, it should be full of love and passion. Right?
Visit site:https://www.queenofindia.in/location/call-girls-in-noida
Your post is very helpful and your information is reliable. I am satisfied with your position. Thank you so much for sharing this wonderful post. Punjabi Bagh Escorts
Assignment Help is a professional academic writing service that provides students with high-quality, plagiarism-free assignments on a variety of subjects. Our team of experienced writers is dedicated to helping students achieve their academic goals and succeed in their studies.
We offer a wide range of Assignment Writing Service, including essays, research papers, term papers, dissertations, and more. We can also help with specific assignments, such as case studies, lab reports, and presentations.
Our assignment writing services are affordable, reliable, and confidential. We understand the importance of deadlines, and we always deliver our assignments on time. We also offer a satisfaction guarantee, so you can be sure that you will be happy with your assignment.
Students start to panic when asked to create UML Diagram Assignment Help because most of them are unfamiliar with the software and algorithms involved. Students frequently experience disappointment when they do not receive the grades they had hoped for. As a result, students can benefit greatly from seeking professional UML diagram assignment assistance. The experts have years of experience and are aware of the academic requirements for perfect UML diagrams.
Looking for online assignment help in Saudi Arabia? Your search ends here! We offer top-notch academic assistance tailored to students’ needs in Saudi Arabia. Our dedicated team of experts specializes in various subjects, ensuring you receive high-quality and personalized support. With our online services, you can access professional guidance and resources from the comfort of your home. Whether it’s essays, research papers, or complex assignments, we provide timely help to meet your deadlines. Our affordable services are designed to alleviate your academic stress, allowing you to focus on learning.
The demands of online classes can be overwhelming. If you find yourself struggling to balance coursework with other responsibilities, online class helper your online class might be the solution. Experienced professionals can attend lectures, complete assignments, and even take exams on your behalf. This service allows you to focus on what truly matters while ensuring academic success. With reliable assistance, you can conquer your online classes with ease and reach your educational goals. So, don’t let the burden of online coursework hold you back; consider paying a trusted expert to manage your classes for you.
Chandigarh call girls
As someone who believes in celebrating work anniversaries with sincerity, I can’t thank this collection of work anniversary messages enough for providing the right words to convey my best wishes.
It is the kind of information I have been trying to find. PG Thank you for writing this information. It has proved utmost beneficial for me.
Thank you for sharing the article. post more often.
pakistani boutique near me
I appreciate you sharing this fantastic website.
Mosque Donation kiosk
Great post! It’s fantastic to learn that Assignment Help Pro is the premier provider of Assignment Help Online in Canada. In today’s digital age, online assistance is invaluable for students, and having a trusted source like Assignment Help Pro is a real boon. Their commitment to aiding students is commendable, especially in a time when remote learning and digital support are more important than ever. With their expertise and reliability, students can confidently seek help with their assignments, knowing they are in capable hands. Thanks for sharing this important information about Assignment Help Pro, a go-to resource for online academic support in Canada!
This is a very useful article and only a few websites on the internet provide such valuable content. I would love to find more of this kind of content from your end.
You suggested this perfectly! thank u.
Add the Hulu Watch Party Chrome extension to your browser for an awesome experience of watching your favorite shows /movies with friends and family in sync with video playback, group chat, and video/audio call features. Share the excitement together!
Are you searching for the best YouTube downloader mp4? then, don’t worry and install our browser extension and download Youtube videos in MP4 format and enjoy hassle-free content. This is the best extension and free to use that allows you to have your favorite shows in MP4 format.
Wer liebt es nicht, watching together movies und mit einer Watch Party eigene Erinnerungen zu schaffen? Sie können jetzt Ihre Lacher,Emotionen und Momente mit Funktionen wie Chat, Audio- und Videoanrufen teilen. Es ist die beste Erweiterung, mit der Sie Spaß haben können.
You suggested this perfectly! thank u.
Thanks for such an valuable information you shared with us. Keep posting.
Visit : Maharaja Matka
Overwhelmed with assignments? Let us handle it for you! Our “Do My Assignment” service takes the stress out of your academic workload. Whether it’s essays, research papers, or any coursework, our professional team ensures high-quality, timely submissions. Focus on what matters most to you, and leave the assignments to us. Relax and let us do the work – contact us today!
Understanding HP printer error codes is crucial for seamless printing experiences. Each code signifies a specific issue, enabling quick diagnosis and resolution. From common errors like ‘Error 0x00759C98’ to more complex ones, knowing these codes empowers users to troubleshoot effectively. Stay informed, and if you encounter any error, refer to the manual or online resources. Being aware of these codes ensures efficient problem-solving, minimizing downtime, and ensuring your printer operates at its best.
This is a great opportunity to visit such a site and I’m glad to know about it. Thank you for giving us the opportunity to take advantage of this opportunity furniture in Pakistan
Very nice blog and post, I found it informative and useful, thank you for kindly share it with us, Unleash the rebel within – our sleek leather jackets redefine coolness and elevate your fashion game effortlessly red puffer jacket
Risk Management Assignment Help is an integral part of various courses, from business to finance and engineering. These assignments are designed to test your ability to assess, analyze, and mitigate risks effectively. However, they can be complex and time-consuming, leaving students feeling overwhelmed.
I found it worth reading please write more about Studed Bracelet
Elevate your living space with the finest furniture from CashAndCarryBeds. Discover unmatched comfort and style today! italian lounge
Unlock exclusive savings at CouponsBliss with our latest coupon codes, where discounts meet convenience for savvy shoppers! student computers discount code
JoinDash invites you to register and unlock the opportunity to earn an incredible 100% commission with our licensed real estate brokerage program. Get started now to maximize your earnings in the real estate industry. flat fee broker for texas
Facing Canon printer error codes? Fret not! Our expert technicians specialize in diagnosing and resolving all Canon printer errors swiftly. Whether it’s error code 5100, 5200, or any other, we’ve got you covered. With our in-depth knowledge and advanced tools, we ensure prompt and effective solutions. Say goodbye to printing hassles! Contact us now, and let us troubleshoot your Canon printer errors, ensuring seamless printing experience. Your satisfaction is our priority, and we’re here to make your printer headaches disappear!
Assignment Help Online offers students a lifeline for their academic challenges. With expert assistance, it simplifies complex tasks, boosts grades, and relieves the stress of looming deadlines. Whether it’s research papers, essays, or any coursework, Assignment Help Online is a trusted resource for academic success.
Brother Auto Parts offers used drive shaft for sale “in the USA”. With our team, you will receive top-quality used drive shafts. Once the drive shafts have made a safe drive, please contact to our team and take these easy steps.
I have read your blog. Your blog is very interesting.
If you are struggling with your Dissertation Writing then do not worry,
Get Dissertation Help UK from Instant Assignment Help.
I love how you presented both sides of the argument and allowed the reader to form their own opinion. This is such an important topic and you handled it with tact and professionalism. Avianca Telefono Miami
where plaque and tartar are removed to prevent gum disease, as well as fillings to address cavities caused by tooth decay. Tooth extractions may be necessary for severely damaged teethfresh dental care
Looking for the right place to get dissertation help? You’re on the same boat as thousands of students who seek out professional assistance with their assignments. The good news is, we are here for you! The dissertation help london
from our website is tailored to enhance your experience and aid you in completing all sorts of academic assignments, whether it be an essay, report or dissertation.
Very Nice Blog! Nursing Assignment Help is the helping hand every nursing student needs. Their unwavering support and dedication to our success have been a constant source of motivation and achievement.
For More Information Visit:Nursing Assignment Help
We offer 40K+ complete accounting assignment help solutions to students at 50% off, and they score A+ grades. Grab the amazing offers before it ends
Business Finance Assignment Help is a vital resource for students seeking guidance in mastering financial concepts and tasks. These services offer expert assistance in areas like financial analysis, budgeting, and investment strategies. They ensure that students receive comprehensive support, enabling them to excel in their assignments and build a strong foundation in business finance. It’s a valuable tool for academic success in this complex field.
In today’s fast-paced digital education landscape, online learning demand has surged, requiring convenience and flexibility. Many students now juggle multiple online classes, causing stress and time constraints. The phrase Pay Someone to Do My Online Class reflects the trend of outsourcing coursework for a balanced approach to education.
There are a array of good-looking girls waiting for you who can assist you to experience the time of your life just by paying a small sum of money. If you are looking for a lover like experience then in that case you can choose these stunning escorts and pamper yourself amazing services. Visit our link to know more https://chandigarhcallgirldehradun.bigcartel.com/
The best faux leather jackets are the ones that make you happy. And our effort is to provide you with faux red leather jacket. So visit our site now.
Need help with your installing netgear wifi extender? We’ve got you covered! Our expert team is here to assist you in setting up and optimizing your extender for seamless, high-speed internet coverage. Say goodbye to dead zones and enjoy a strong WiFi signal throughout your home or office. Contact us by phone at +1-323-471-3045 or by email at support@mywifiextension.net.
I have read your blog. Your blog is very interesting.
If you are struggling with your Dissertation Writing then do not worry,
Get Dissertation Help UK from Instant Assignment Help.
Marketing fundamentals form the cornerstone of any successful marketing strategy. When students seek marketing fundamentals assignment help, they acquire a solid foundation in key marketing principles, such as market segmentation, target audience analysis, product positioning, and the marketing mix (4Ps – product, price, place, and promotion). This guidance helps students comprehend the essential elements that underpin marketing strategies, enabling them to develop effective marketing plans and campaigns that connect with consumers and drive business success.
Many people have worry lines on their foreheads from being unable to set up their Netgear EX6120 extenders. This manual is for you if you are also unable to proceed with the process. Not one, not two, but three distinct approaches of doing Netgear EX6120 setup have been discussed here. So stop waiting and carry on reading.
If you have any issues with your extender , you can contact our professional expert or dial our toll-free number (+1-323-471-3045) or email: support@mywifiextension.net
It’s really great. Thank you for providing a quality article. There is something you might be interested in. Check out our Winter training in Noida as well. If you are having trouble finding SAS Training in noida. Thus, don’t hesitate any longer and use our services.
It’s really great. Thank you for providing a quality article.
If you want to meet sexy girls at our desired location then click on this link location and hire sexy girls in Mukherjee Nagar (Delhi).
Mukherjee Nagar Escorts
This is extremely glorious stuff for me. I got information from your blog. keep sharing
As a professional content writer with a bachelor’s degree in health and economics, she specializes in gathering details, checking facts about foods that are diuretics! and making complex subjects easy to understand. foods that are diuretics In addition to writing articles, foods that are diuretics! about foods and drinks that are natural diuretics. I am awesome at writing healthcare news, abstracts, journals, and blogs.
This reputed educational organization from Bangalore was in need of a brand identity that aptly captured their legacy, their strength and their value proposition. So we combined three elements to tell their story. A rising sun signifies a bright future or a new dawn for their students, a tree signifies growth, and a book to signify knowledge. The logo was given a traditional monogramesque look but in a modern style to bridge the past with the future.
Specializing in sensor technology, our profile on Exporthub.com within the B2B domain showcases our expertise in Rectangular Infrared Photoelect Sensors. With a focus on precision and functionality, our sensors are designed to detect various objects accurately. Our range caters to diverse industries, ensuring reliability and efficiency in automated systems. We prioritize quality, offering sensors built with advanced technology to meet the specific needs of our clients.
Farewell Card For Coworker are the poetic and artistic expressions of our love and good wishes, serving as both a source of comfort and inspiration during times of transition.
I am a very simple and cute girl with a lot of love and working in Green Park Escorts Agency, or you can say Escort in Green Park.
Our Green Park Escorts services are available 24*7 to make your love life happy. Yes, I am serving as an affordable Escort service in Green Park.
Crafting a robust Sop-for-Industrial-engineering involves delineating a vision that merges innovation and efficiency. It’s not just a document; it’s a blueprint for optimizing processes and pioneering advancements in industrial landscapes.
This presents a wonderful opportunity to explore such a website, and I’m delighted to have come across it. I appreciate you affording us the chance to make the most of this offer Best Air duct cleaning service in Florida
Finance Assignment Help provides invaluable assistance to students navigating complex financial concepts and assignments. The service ensures a deep understanding of topics like financial management, investment analysis, and risk assessment. Expert tutors offer personalized guidance, fostering academic success and confidence in financial studies. A reliable resource for achieving excellence in finance education.
Izel Apparel is a distinguished women’s clothing brand that exudes elegance and style. With a keen eye for fashion trends and a commitment to providing high-quality attire, Izel Apparel offers a diverse range of clothing that caters to the modern woman’s wardrobe needs. From chic dresses and versatile separates to comfortable loungewear, Izel Apparel seamlessly combines fashion-forward designs with superior craftsmanship, ensuring that every piece not only looks great but also feels exceptional to wear. Whether you’re dressing up for a special occasion or seeking everyday fashion staples, Izel Apparel is your go-to destination for sophisticated and on-trend women’s apparel.
This presents an amazing chance to investigate this website, and I’m thrilled to have found it. I value the opportunity you’ve given us to take full advantage of this offer Dry vent cleaning service in florida
When your Canon printer shows as offline, it means that it is unable to communicate with the computer, hindering the printing process. This issue can occur due to various factors, including connection problems, outdated drivers, or misconfigured settings. Before attempting any fixes, it is essential to determine the root cause of the problem.
The advent of online gaming platforms has brought crash games to a global audience. crash games online provide players with the convenience of accessing these adrenaline-pumping experiences from the comfort of their devices. These platforms often feature user-friendly interfaces, a variety of crash game variants, and real-time multiplayer options. Players can enjoy the suspense of watching multipliers rise and strategically decide when to exit the game to maximize their winnings, creating a dynamic and engaging online gaming experience.
Looking for a lawyers for bankruptcies near me,Our skilled lawyer will provide a legal advice to you.
Practice more than 10 sets of JEE Main Mock Test at Mockers for free. The mock tests are based on the latest exam pattern prepared by subject experts for the ease of aspirants. Taking the JEE Main Mock Test regularly will help you assess your preparation level and learn to manage time to score higher marks in the Engineering entrance test.
Practice more than 10 sets of JEE Main Mock Test at Mockers for free. The mock tests are based on the latest exam pattern prepared by subject experts for the ease of aspirants. Taking the JEE Main Mock Test regularly will help you assess your preparation level and learn to manage time to score higher marks in the Engineering entrance test.JEE Main Mock Test
2024
Best Event Planner & Wedding Planner in Jaipur, India. With our top event planners we give you an amazing wedding experience to make your dream wedding come true
This presents an amazing chance to investigate this website, and I’m thrilled to have found it. I value the opportunity you’ve given us to take full advantage of this offer chimney cleaning service in florida
This Assignment Help blog is a valuable resource for students navigating the complexities of academic tasks. The insightful content covers a spectrum of subjects, offering not just solutions but a deeper understanding of the topics. The emphasis on quality, timely delivery, and personalized assistance is commendable. Reading through, it’s evident that this service goes beyond mere assignment completion; it fosters learning and skill development.
This is a very nice blog for you all the information given here is so useful and as per the requirements keep on posting like this thanks.
Mukherjee Nagar Escort
This presents an amazing chance to investigate this website, and I’m thrilled to have found it. I value the opportunity you’ve given us to take full advantage of this offer air duct cleaning service in florida
Appreciation for the delightful post! Your commitment and effort are truly impressive. The perfect destination for escalating traffic to your webpages. Love it! see more
Your search for the ultimate gaming destination ends at betflix168 เข้าสู่ระบบ Play now, win big!
Hii, Admin
Thanks for sharing with us!!
https://thegodrejproperties.net.in/
Python backtesting libraries play a pivotal role in the financial realm, enabling traders and analysts to evaluate the performance of trading strategies in a simulated environment. These libraries provide a robust framework for testing strategies against historical market data, helping users make informed decisions and refine their approaches. Notable libraries like Backtrader, PyAlgoTrade, and QuantConnect offer a range of features, from customizable trading logic to detailed performance metrics. The Python ecosystem’s flexibility and extensive community support further enhance the appeal of these libraries, making them valuable tools for both beginners and seasoned professionals in the world of algorithmic trading. Most students are drawn to these types of articles and information, but they are unable to prepare for their exams, If you have been struggling with your exams and want assistance, students can do my exam – do my exam for me and get higher grades on their examinations by providing them with the best available resources, including quality academic services.
College Assignment Help in Canada is a reliable resource for students seeking assistance with their assignments. With expert guidance and personalized support, it helps students achieve academic success and overcome challenges in their college coursework.
Anju Rana is a brand name. She is offering genuine escorts in Delhi. She have an excellent reputation with t heir clients. Because their escort girls take escort service experience to the next level, nobody can beat our service quality. For the last five years, we have been the number-one escort agency in Delhi.
This presents a wonderful opportunity to explore such a website, and I’m delighted to have come across it. I appreciate you affording us the chance to make the most of this offer Dry vent cleaning service in florida
sun country change fee
Welcome to Tanu Rao Delhi Escorts Service, located in the exotic state of India. Our dedicated team of sexy delhi escort girls is hand-picked with care & their services come in various options and pricing deals to suit any budget.
This provides an excellent chance to discover the website, and I’m excited to have stumbled upon it. Appreciate the chance to make the most of this offer chimney cleaning service in florida
Imagine stepping into a world where every dish tells a story and every bite ignites a burst of flavors. Whether you’re a fan of fiery curries, aromatic biryanis, or mouthwatering kebabs, you’ll find it all in the rich tapestry of Indian cuisine. From the tangy street food of Mumbai to the royal feasts of Rajasthan, Indian food is Dinner Restaurant in Temple Tx a true culinary adventure. In this blog, we’ll take you on a journey through the diverse and delicious world of Indian cuisine.
I really amazed to read this blog post. It is so unique and informative
Hello call girls in Delhi searchers, I am Ananya Chopra one of the attractive and professional Female. If you are searching for a true partner and want to spend your private time together that is deeply satisfy you, then most welcome you to our websites.
This provides an excellent chance to discover the website, and I’m excited to have stumbled upon it. Appreciate the chance to make the most of this offer best vent cleaning service in florida
Dissertation Help offers crucial guidance to scholars embarking on extensive research projects. Expert advisors provide invaluable support in refining research methodologies, structuring arguments, and ensuring academic rigor. This service streamlines the complex process, fostering clarity and originality in scholarly work, ultimately leading to a well-crafted and impactful dissertation.
IT Assignment Help connects you with professionals who have a deep understanding of IT principles and the latest industry trends. IT assignment help offers personalized solutions, ensuring your work is relevant to your coursework.
This offers a fantastic opportunity to explore the website, and I’m thrilled to have come across it.
Grateful for the opportunity to take advantage of this offer best chimney cleaning service in florida
Khelraja goes the extra mile in providing an enticing opportunity for players to kickstart their gaming journey without the need for an initial deposit. Offering some of the Best Online Slot Games Real Money with no deposit, the platform welcomes players with open arms, allowing them to experience the thrill of winning without risking their own funds.
Online Class Help USA is a leading and popular platform for online class assistance. Get reliable and professional Online Class Help services to excel in your courses. Our experts offer personalized assistance and ensure your academic success.
I am genuinely grateful for your informative post! Your shared insights on assignment writing services in Australia are incredibly valuable. The reassurance that one can reach out to your expert team at Assignment Help in Australia
is especially comforting for those facing academic challenges. Your commitment to offering professional assistance is evident and commendable. Thank you for not only providing crucial information but also extending a lifeline for individuals navigating the complexities of assignments. Your post serves as a beacon of support, offering a reliable solution to those in need. It’s contributions like these that make a positive impact on the academic journey.
Mymathlab Answers is a leading and popular platform for Mymathlab assistance. We are the leading MyMathLab services and offer reliable MyMathLab answers. Our experts are keenly available to serve every student in the best possible ways. We are committed to affordably providing math lab solutions.
A CTET national-level test is conducted by the Central Board of Secondary Education (CBSE). CTET exam is held to check students’ eligibility for the post of teacher in Central Government Schools such as KVS, NVS, and others. To prepare well for the entrance exam, students can solve the CTET Mock Test 2023 as it involves various kinds of questions.CTET Mock Test 2023