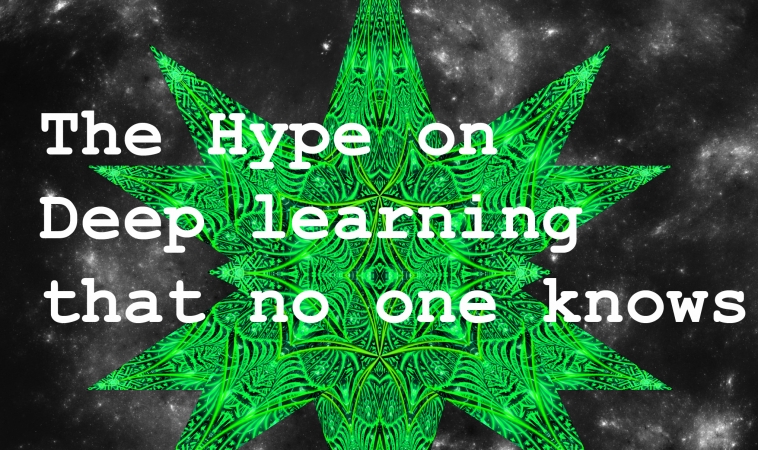
Hype on Deep Learning That No One Knows
The techniques developed from deep learning research during the last decade have impacted a broad range of signal and information processing work. It has widened scopes including key aspects of machine learning and artificial intelligence.
Deep learning is a very broad field. It exceeds far ahead than the general perception that people have about Visual Recognition, Speech to Text or Natural Language Processing. It encompasses wide-ranging machine learning techniques, while making the best use of non-linear information which is hierarchical in nature. For a flavor of possibility, let us see the commercial uses of deep learning applications.
- Agriculture: One would think of the role that deep learning has to play in the field of agriculture and farming. This modern era has replaced the traditional methods and has made the agriculture process and farming mechanized. Deep learning has introduced the concept of; Satellite Images for better management and improved response time, use of sensor data in order to have better farming techniques incorporated and learn from the past trends by analyzing series of data, and lastly the most important; the seed genetics as it helps to improve the quality of the crop analyzing the fertility of the soil. Deep Learning has eliminated the element of experiment by providing a set method of analysis and outcomes based on learning.
- Finance: Over the last few decades, the modernization in the finance sector has been astonishing. Ledger books being replaced by amazing software while ensuring accuracy. Economic and financial Modeling has become a significant part for Government Institutes and International firms. These computer models have set a new standard in the field of deep learning. All you have to do is input a certain set of data into the neural network and just allow it to make predictions for the future, based on past trends. Deep Learning has taken it a step further with advancements in Time-series and Econometric Analysis. You just regress the data and you are all set for economic and financial forecasting.
- Biology: Advancements in the field of medicine and biology have been truly revolutionary. Tremendous amounts of machine / statistical data used in research can be analyzed faster than ever. Machine Learning has increased the pace of medical discoveries, and at the same time has introduced life saving techniques. Discoveries in DNA, drugs and genetic modification have taken biological sciences to a whole new level.
- Radiology: Medical imaging has made life easier for humans. Deep learning can be used to create heat maps on the medical images and to highlight the areas of interest. Just imagine the level of efficiency and accuracy in CT scans, MRI and ECG which can be attained through deep learning, and at the same time making the data set analysis in radiology even simpler.
- Video Recognition: The amount of video data over the internet is massive. It might be required to make it discrete according to consumer preferences. Deep Learning facilitates with the help of algorithms to analyze and use the video better than ever before. Deep Learning has improved the analysis of security camera footage and in extracting the information from online videos.
Over the period of time, and depending on how deep learning techniques are intended to be used, it can be broadly categorized into three major classes.
- Deep networks for unsupervised learning, are intended to capture high-order correlation of the visible data for analyzing the patterns when no information about target class labels is available. The “unsupervised feature” in literary terms refers to this class of the deep networks. While being used in the generative mode, this may also be intended to characterize joint statistical distributions of the visible data along with their associated classes when available and being treated as part of the visible data.
- Deep networks for supervised learning, are anticipated to directly provide discriminative power for pattern classification purposes. This is done by characterizing the subsequent distributions of classes conditioned on the visible data. Target label data are always available in direct or indirect forms for such supervised learning. They are also called discriminative deep networks.
- Hybrid deep networks, their main goal is discrimination which is assisted, often in a significant way, while using the outcomes of generative or unsupervised deep networks. This can be accomplished by better optimization of the deep networks in the previous category.
Deep learning has gained importance over the years. If we talk about the year 2006 and before that, deep learning and relevant architectures were unsuccessful. Primarily due to less investigations in this field. However, things have changed now. All thanks to;
- Faster machines and more data helps DL more than other algorithms
- New methods for unsupervised pre-training have been developed (Restricted Boltzmann Machines = RBMs, auto encoders, contrastive estimation, etc.)
- More efficient parameter estimation methods
- Better understanding of model regularization, ++
What can be better than having data driven medicine to support the existence of the human race, economic and financial performances to forecast state stability and getting desired information from clustered data. Deep Learning makes it all and much more possible.
Greater accuracy in classifying data enables us to make more informed decisions according to one’s own personal requirements. Deep learning delivers accuracy, but eventually it still does depend on us in making good decisions.
Brought to you by the RobustTechHouse team (A top app development company in Singapore). If you like our articles, please also check out our Facebook page.